Filtrar por género
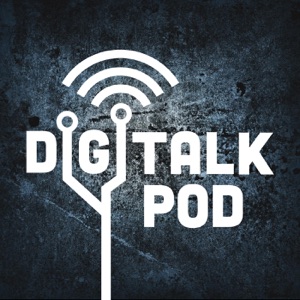
- 11 - 11. Knowledge creation for businesses in the digitalization era
Guest: Robin Teigland, Professor in Management of Digitalization in the Entrepreneurship and Strategy Division at Chalmers University of Technology, Department of Technology Management and Economics.
Quote: “Digitalization challenges many of the basic assumptions around the way business is being conducted for “.
Check out Robin’s publications:
Ingram Bogusz, C., Teigland, R., & Vaast, E. (2019). Designed entrepreneurial legitimacy: the case of a Swedish crowdfunding platform. European Journal of Information Systems, 28(3), 318-335. https://orsociety.tandfonline.com/doi/full/10.1080/0960085X.2018.1534039#.XP168YgzaUk
Felländer, A., Ingram, C., & Teigland, R. (2015). Sharing economy. In Embracing Change with Caution. Näringspolitiskt Forum Rapport (No. 11) https://www.researchgate.net/profile/Robin_Teigland/publication/278410531_SHARING_ECONOMY_EMBRACING_CHANGE_WITH_CAUTION/links/5580805808aed40dd8cd27ee.pdf
Wasko, M. M., Faraj, S., & Teigland, R. (2004). Collective action and knowledge contribution in electronic networks of practice. Journal of the Association for Information Systems, 5(11), 15. https://aisel.aisnet.org/jais/vol5/iss11/15/
Tue, 11 Jun 2019 - 32min - 10 - 10. Smart Manufacturing: a Simulation-based Perspective
Guest: Sanjay Jain, Associate Professor of Decision Sciences at George Washington University, Washington, DC and research associate at NIST (National Institute of Standards and Technology) in the system engineering group.
“You have to make the [artificial] intelligence accessible and easy to use for the industry”
We recorded this episode in December 2018 in the venue of the Winter Simulation Conference, which was hosted in Gothenburg, and attracted +1000 between speakers and participants. Our guest, Sanjay Jain, was the program chair of the conference. Sanjay was able to bring a different perspective on industrial digitalization from the perspective brought by our previous guests, who were all based in Europe. In fact, Sanjay is based in the US and works in a multi-faceted environment: academia, a governmental institution, and in a business school, holding MBA classes.
What does the Industrial Internet of Things mean when put into the context of Smart Manufacturing? Sanjay Jain breaks it down in three pillars, following McKinsey’s classification: connectivity, intelligence and automation, where all three have to come together and work in unison. Sanjay explains that if the intelligence that smart-manufacturing applications is not accessible and easy to use for the industry, the increased effort for business people in using those applications will make their value plummet, which would cripple the research investment being put into those. This applies to SMEs in particular.
Among connectivity, intelligence and automation, Sanjay focuses on the intelligence part, applied at a production-system level. In his latest article, Sanjay and his co-authors built a simulation model to quickly estimate cycle times for incoming orders for promising delivery dates. Within the multitude of data-analytics approaches and machine-learning techniques, choosing the best approach/technique to estimate these cycle times is a tough job, with lots of uncertainty. Plus, enough real data from the production system is lacking and it is not enough to feed the algorithms properly. In his paper, two approaches, Neural Networks (NN) and Gaussian Process Regression (GPR), are evaluated using data generated by a manufacturing simulation model itself, skipping the need for the use of lots of real data from the production system. The results showed that the GPR model performed well when trained using limited data and also when the factory is operating under the high load condition.
Sanjay Jain, Associate Professor of Decision Sciences at George Washington University, Washington, DC and research associate at NIST (National Institute of Standards and Technology) in the system engineering group.
Check out Sanjay’s publications:
Jain, S., Anantha Narayanan, A., Yung-Tsun, T.L COMPARISON OF DATA ANALYTICS APPROACHES USING SIMULATION. In Proceedings of the 2018 Winter Simulation Conference. https://www.informs-sim.org/wsc18papers/includes/files/090.pdfTue, 09 Apr 2019 - 24min - 9 - 9. Assessing Smart Maintenance
Guest: Jon Bokrantz, PhD student, Chalmers University of Technology, Division of Production Systems, Department for Industrial and Materials Science
“This shared understanding of smart maintenance is rooted in the Swedish manufacturing industry. They really defined what it is”.
Jon stated: “We cannot build factories that are highly dependent on very advanced manufacturing technology and then allow them to stand still half of the time.” Jon explained the delicate interplay between maintenance management and competitiveness of manufacturing Jon pinpointed the importance of building a unified language to describe the pillar-concepts of smart maintenance. Not only for researchers to work smoothly with practitioners and viceversa. Jon described Smash – assessment of smart maintenance. The aims to SMASh project was to enable digitalization of the Swedish manufacturing industry. Many different management roles were involved in the development of the assessment tool.Check out Jon’s publications:
Bokrantz, J., Skoogh, A., Berlin, C., & Stahre, J. (2017). Maintenance in digitalised manufacturing: Delphi-based scenarios for 2030. International Journal of Production Economics, 191, 154-169. https://doi.org/10.1016/j.ijpe.2017.06.010
Ylipää, T., Skoogh, A., Bokrantz, J., & Gopalakrishnan, M. (2017). Identification of maintenance improvement potential using OEE assessment. International Journal of Productivity and Performance Management, 66(1), 126-143. https://doi.org/10.1108/IJPPM-01-2016-0028
Bokrantz, J., Skoogh, A., Berlin, C., & Stahre, J. (2017). Maintenance in digitalised manufacturing: Delphi-based scenarios for 2030. International Journal of Production Economics, 191, 154-169. https://doi.org/10.1016/j.ijpe.2017.06.010
Fri, 29 Mar 2019 - 24min - 8 - 8. The Digital Twin for Geometrical Variations Management 4.0
Guest: Professor Rikard Söderberg, Chalmers University of Technology, Head of Department of Industrial and Materials Science and Director of Wingquist Laboratory.
“If we feed our simulation algorithms with real-time data, we can compensate [geometrical deviations] and optimize the production”.
Professor Rikard Söderberg takes us to a journey from the dawn of the engineering discipline of geometrical assurance to the digital twin as key to manage product tolerances and adjust the production according to the varieties of the upcoming products.
The goal? Reduce costs, waste, and improve quality. Speaking about research utilization and marketable innovation, Rikard invites current budding researchers-entrepreneurs to keep their vision tight and to work hard in a journey that can last for up to 20 years.
• Rikard introduces the episode by talking about how his different professional roles act in synergy. He also stressed the importance of focus, vision (personal effectiveness) and elimination of time waste (personal efficiency).
• Rikard Söderberg continues with the history of geometrical variation and geometrical assurance as engineering discipline in manufacturing/production research. Despite this discipline being quite “traditional”, he stressed the importance of bringing it to the industrial practice in order for companies to achieve cost reduction and value-creation opportunities in terms of quality, both “real” and “perceived”.
• Rikard explained the alternatives of securing quality upfront by putting proper tolerances on key features of the product (the stricter tolerances are, the more expensive they will be), as opposed to be “more relaxed” in product design but run higher quality-related and functionality-related risks during the use phase of the product. Software packages can help companies manage this trade off in early product development phase.
• Rikard introduced the concept of the digital twin, a digital copy of the real world product. This digital twin of the product “works” through simulation algorithms that are fed with real-time data from the shop floor. The digital twin needs to be precise and accurate. But, again, what’s good enough? Can we scan same takt time as the production line?
• Rikard talks about the “reparatory” power of software programs combining analytics and production simulation that compensate in production what goes wrong as the product gets manufactured. However, calculations of these “compensations” need to be made in the same takt time of the production line, and this is a challenge. Opportunities exist, though. Components can be scanned when they leave the supplier’s site.
• In relation to the software packages with the capabilities described above, Rikard added that the tougher the competition is in the market for manufacturers, the higher the need for tools for tolerancing is.
• Speaking about innovation, Rikard invites current budding researcher-entrepreneurs to keep their vision tight and to work hard. Results can show up in up to 20 years.
• A final line from Rikard: “Coming from product development, the final validation is when somebody buys your product”.
Check out some of his publications:
Rikard Söderberg, Kristina Wärmefjord, Julia Madrid, Samuel Lorin, Anders Forslund, Lars Lindkvist. An information and simulation framework for increased quality in welded components. CIRP Annals, Volume 67, Issue 1, 2018
Edward Morse, Jean-Yves Dantan, Nabil Anwer, Rikard Söderberg, Giovanni Moroni, Ahmed Qureshi, Xiangqian Jiang, Luc Mathieu. Tolerancing: Managing uncertainty from conceptual design to final product. CIRP Annals,Volume 67, Issue 2, 2018, Pages 695-717.
Rikard Söderberg, Lars Lindkvist, Kristina Wärmefjord, Johan S. Carlson, Virtual Geometry Assurance Process and Toolbox. Procedia CIRP, Volume 43, 2016, Pages 3-12,
Wed, 13 Mar 2019 - 26min - 7 - 7. Big Data for Big Decisions in Maintenance
Guest: Mukund Subramaniyan, PhD student, Chalmers University of Technology
“The data speaks about the behavior of the production system”
Instead of defining big data in terms of “what” and “how”, Mukund Subramaniyan invites us to asks: “why” big data? In this episode, Mukund Subramaniyan shares his adventure and precious knowledge as cross-disciplinary PhD student, bridging the gap between computer science and production engineering. His motto? Big data for big decisions.
Mukund sees the potential of data in the production system, and uses his mathematical skills, combined with his knowledge about production systems’ operations, to find the most efficient and effective way to transform data into knowledge. His mission is to help managers and engineers in the production and maintenance departments to make more accurate decisions with higher degree of confidence.
Mukund’s position in terms of balance between automation and human’s contribution is that algorithms should be giving an augmented intelligence to humans as opposed to be the representatives of an artificial intelligence that does all the job, simply put. Mukund argues that 60-70 % of the work can be done by algorithms, and the remaining part of the work is up to the humans, who judge the results according to their experience, and make the final decision.
Check out Mukund’s publications:
Subramaniyan, M., Skoogh, A., Salomonsson, H., Bangalore, P., Gopalakrishnan, M., & Sheikh Muhammad, A. (2018). Data-driven algorithm for throughput bottleneck analysis of production systems. Production & Manufacturing Research, 6(1), 225-246.
https://doi.org/10.1080/21693277.2018.1496491
Subramaniyan, M., Skoogh, A., Gopalakrishnan, M., Salomonsson, H., Hanna, A., & Lämkull, D. (2016). An algorithm for data-driven shifting bottleneck detection. Cogent Engineering, 3(1), 1239516.
DOI: 10.1080/23311916.2016.1239516
Thu, 28 Feb 2019 - 25min - 6 - 6. Optimal Factory Layout thanks to Virtual Reality
Guest: Liang Gong, PhD student, Chalmers University of Technology.
“With a realistic model of the factory [in VR], actors affected by changes in factory layout are actively involved in the planning process”.
Liang Gong is a PhD student at the division of Production Systems at Chalmers University of Technology, and is the expert of Virtual Reality technologies adoption in his research group. Liang explained how computer aided design (CAD) and simulation tools are great for estimating quantitative measures in factory layout planning (such as travel distance from A to B). However, such traditional approaches do not provide rich insights for detailed layout planning, where qualitative factors such as safety and ergonomics are key. Liang, in his three years of research at Chalmers, carried out three empirical studies in the automotive industry, where he asked managers to evaluate different VR integration approaches. Thanks to those studies, Liang came up with general guidelines on how to integrate and use virtual reality technology for factory layout planning.
Check out Liang’s publications:
Gong, L., Berglund, J., Saluäär, D., & Johansson, B. (2017). A novel VR tool for collaborative planning of manufacturing process change using point cloud data. Procedia CIRP, 63, 336-341. https://doi.org/10.1016/j.procir.2017.03.089 Berglund, J., Gong, L., Sundström, H., & Johansson, B. (2017). Virtual Reality and 3D Imaging to Support Collaborative Decision Making for Adaptation of Long-Life Assets. In Dynamics of Long-Life Assets (pp. 115-132). Springer. https://link.springer.com/chapter/10.1007/978-3-319-45438-2_7#Bib1Wed, 23 Jan 2019 - 13min - 5 - 5. Utilizing Data from the Production System
This is a special episode, because Maja Bärring and Daniel Nåfors are the first PhD students who have been interviewed in Digitalk Pod. Maja’s research focuses on understanding the value of data brought by digital technologies in production, such as the 5G and blockchain, whereas Daniel’s research focuses on supporting layout planning in factories via 3D laser scanner technology applied to virtual reality.
Daniel and Maja started by telling why they left their former thriving employer companies (Maja worked at Siemens and Daniel worked at Volvo Trucks) to become PhD students. The driving force was the possibility to understand complex phenomena and thanks to such an understanding, a contribution to innovate production systems more rapidly.
Then, Daniel and Maja illustrated the key activities of and findings from the research project which contributed to their education the most.
For Maja, the 5GEM (5G-enabled manufacturing) project was key. This project generated in SKF five pilots that demonstrate the use of advanced and mobile communication provided by the 5G network. Ericsson assisted with his telecommunications capabilities. A specific challenge was using the data in standardized and interoperable format when connecting machines, products and humans’ activities in the shop floor.
For Daniel, the key project was 3D silver. The project provided the decision makers in the manufacturing industry with the technology enabled by point cloud data, 3D imaging, to facilitate layout planning with the highest accuracy possible and therefore avoiding costly mistakes.
Check out Maja’s and Daniel’s publications:
Bärring, C. Lundgren, M. Åkerman, B. Johansson, J. Stahre, U. Engström, and M. Friis. ”5G Enabled Manufacturing Evaluation for Data-Driven Decision-Making”. Procedia CIRP 2018. Volume 72, pp. 266-271, https://doi.org/10.1016/j.procir.2018.03.169.Dsda Nåfors, E. Lindskog, J. Berglund, L. Gong, B. Johansson and J. Vallhagen, "Realistic virtual models for factory layout planning," 2017 Winter Simulation Conference (WSC), Las Vegas, NV, 2017, pp. 3976-3987. doi: 10.1109/WSC.2017.8248107Wed, 19 Dec 2018 - 18min - 4 - 4. The Future of Maintenance
Guest: Anders Skoogh, Associate Professor and research group leader for Production Service Systems & Maintenance, Department of Industrial and Materials Science, Chalmers University of Technology.
“The vision has to be failure-free production”
Listen to Anders Skoogh, who brings a perspective that combines technology, management and strategy and that transforms the concept of maintenance from reactive and insular to proactive and collaborative. Anders, being the Director of the Production Engineering Master Programme, describes how digitalization has been brought into curricula.
Some of the topics that Anders illustrated during the conversation:
The Overall Equipment Effectiveness, a pivotal indicator in maintenance management. If you want to know more, check this article by Torbjörn Ylipää, Anders Skoogh, Jon Bokrantz, Maheshwaran Gopalakrishnan, (2017) "Identification of maintenance improvement potential using OEE assessment", International Journal of Productivity and Performance Management, Vol. 66 Issue: 1, pp.126-143, https://doi.org/10.1108/IJPPM-01-2016-0028 The shift from condition-based maintenance to predictive-based or even prescriptive-based maintenance: in the future, failures will be predicted and the most efficient course of action will be advised. The technology that “smartifies” maintenance, e.g., 5G connectivity, sensor systems, integration of data from sensors with Manufacturing Execution Systems (MES) and Enterprise Resource Planning (ERP) The importance of achieving smart maintenance through not only “a bunch of technologies” but also an intentional organizational changes and re-shaping of the organizational competences in manufacturing. Servitization of production services.Wed, 05 Dec 2018 - 28min - 3 - 3. The Digital Factory
Guest: Professor Björn Johansson, Chalmers University of Technology
“The digital factory is our laboratory… [whose] data comes from the real world”.
In this episode, Professor Björn Johansson talks about virtual production and digital twins, and shares with us how digital tools (for instance, 3D imaging, discrete event simulation) can help improve the sustainability performance of factories. Björn’s research group aims at improving productivity and the circular-economy performance in not only automotive and aerospace, but also in the maritime, textile and electronics industry. Björn also discussed utilization strategies in research (publishing or patenting?), and the open collaboration pattern between research and academia in Sweden. Björn also remarked how awareness of the production system’s performance and behaviour leads to smarter decisions for sustainability. Answering the tricky question about the huge number of jobs’ displacement because of AI, Björn shares his positive outlook about the future, when industrial performance are optimized, people have new types of jobs and more time for leisure.
Read more about:
Björn Johansson, Professor of Sustainable Production at the Department of Industrial and Materials Science at Chalmers. Ecoprodigi, a flagship project to foster eco-efficiency in the Baltic Sea region maritime sector through digital solutions in vessel’s design and ship operations. BOOST 4.0, an EU project that aims at developing and testing models for data-driven Factory 4.0 through ten lighthouse factories. Opportunities of Sustainable Manufacturing in Industry 4.0 by T. Stock and G. Seliger, Procedia CIRP, Volume 40, 2016.Wed, 21 Nov 2018 - 20min - 2 - 2. Sustainable Manufacturing
Guest: Mélanie Despeisse, Assistant Professor, Chalmers University of Technology.
“Manufacturing needs to be efficient but also respect the planetary boundaries”.
In this episode, Assistant Professor Mélanie Despeisse takes us to a journey starting from the quintessence of manufacturing – using valuable resources to produce value for society – to sustainable manufacturing and related concepts: eco-efficiency vs eco-effectiveness, and circular economy, just to name a few. Mélanie also tells us her journey as world-citizen researcher starting in UK, Belgium, Japan and then Sweden. Mélanie continues the chat with examples of digitalization applied for mass customization, additive manufacturing and digitalization opportunities for SMEs. Mélanie concludes the chat with her experience in designing games for teaching sustainable leadership.
Read more about:
Mélanie Despeisse, Assistant Professor of Sustainable Manufacturing at the Department of Industrial and Materials Science in Chalmers Planetary boundaries Eco-efficiency vs eco-effectiveness Teaching sustainability leadership in manufacturing: Factory Heroes board game designed by Mélanie DespeisseWed, 07 Nov 2018 - 33min - 1 - 1. What is Digitalization?
Guest: Professor Johan Stahre, Chalmers University of Technology.
Digitalization tells “how you should use a new technology in order to be more productive, safer, and more creative”.
In this episode, Professor Johan Stahre tells us what digitalization is, and how it is leveraging manufacturing companies’ capabilities to be more productive and more innovative. Johan also tells what the division of production systems at Chalmers is doing in order to build the engineering competences needed for the fourth industrial revolution. Johan also explained what digitalized factories are capable to do. We zoomed in on the 5G network as standard to convey key information. The talk concluded with a mention on the role of the SMEs in the fourth industrial revolution.
Don't forget to subscribe to the show!
Wed, 24 Oct 2018 - 22min
Podcasts similares a DigiTalk Pod
Global News Podcast BBC World Service
Kriminálka Český rozhlas
El Partidazo de COPE COPE
Herrera en COPE COPE
The Dan Bongino Show Cumulus Podcast Network | Dan Bongino
Es la Mañana de Federico esRadio
La Noche de Dieter esRadio
Hondelatte Raconte - Christophe Hondelatte Europe 1
Affaires sensibles France Inter
La rosa de los vientos OndaCero
Más de uno OndaCero
La Zanzara Radio 24
Espacio en blanco Radio Nacional
Les Grosses Têtes RTL
L'Heure Du Crime RTL
El Larguero SER Podcast
Nadie Sabe Nada SER Podcast
SER Historia SER Podcast
Todo Concostrina SER Podcast
安住紳一郎の日曜天国 TBS RADIO
The Tucker Carlson Show Tucker Carlson Network
辛坊治郎 ズーム そこまで言うか! ニッポン放送
飯田浩司のOK! Cozy up! Podcast ニッポン放送
武田鉄矢・今朝の三枚おろし 文化放送PodcastQR