Filtrar por género
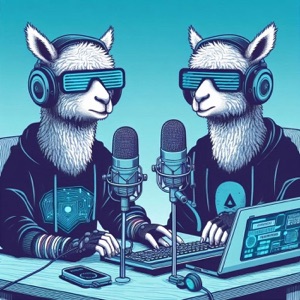
- 49 - Marco-o1
🤖 Marco-o1: Towards Open Reasoning Models for Open-Ended Solutions
The Alibaba MarcoPolo team presents Marco-o1, a large reasoning model designed to excel in open-ended problem-solving. Building upon OpenAI's o1 model, Marco-o1 incorporates Chain-of-Thought fine-tuning, Monte Carlo Tree Search, and innovative reasoning strategies to improve accuracy on complex tasks. The model is trained on a combination of existing and synthetic datasets and shows improvements in accuracy on benchmark datasets, particularly in handling nuanced language translation. Further research focuses on refining the reward system within the Monte Carlo Tree Search and using reinforcement learning to enhance its capabilities. The paper details the model's architecture, training process, and experimental results, highlighting its advancements in open-ended reasoning.
📎 Link to paperSat, 23 Nov 2024 - 48 - Scaling Laws for Precision
⚖️ Scaling Laws for Precision
This research paper investigates the impact of precision in training and inference on the performance of large language models. The authors explore how precision affects the effective parameter count and propose scaling laws that predict performance degradation due to low-precision training and post-training quantization. They find that overtrained models are more sensitive to post-training quantization, and that training larger models in lower precision might be computationally optimal. Their unified scaling law accounts for both training and post-training effects and predicts loss in varied precision settings, ultimately suggesting that the standard practice of training models in 16-bit might be suboptimal.
📎 Link to paper
🌐 Read their TweetMon, 18 Nov 2024 - 47 - Test-Time Training
⌛️ The Surprising Effectiveness of Test-Time Training for Abstract Reasoning
This paper examines how test-time training (TTT) can enhance the abstract reasoning abilities of large language models (LLMs). TTT, which updates model parameters during inference, significantly improves performance on the Abstraction and Reasoning Corpus (ARC) benchmark. Key factors for effective TTT include initial fine-tuning, auxiliary tasks, and instance-specific training. The approach achieves state-of-the-art results on ARC, even matching human averages with program synthesis. This study suggests that dedicating computation at test time, rather than relying on symbolic components, may be essential for complex reasoning tasks.
📎 Link to paperThu, 14 Nov 2024 - 46 - Qwen2.5-Coder
🔷 Qwen2.5-Coder Technical Report
The report introduces the Qwen2.5-Coder series, which includes the Qwen2.5-Coder-1.5B and Qwen2.5-Coder-7B models. These models are specifically designed for coding tasks and have been pre-trained on a massive dataset of 5.5 trillion code-related tokens. A significant focus is placed on data quality, with detailed cleaning and filtering processes, and advanced training techniques such as file-level and repo-level pre-training. The models were rigorously tested on various benchmarks, including code generation, completion, reasoning, repair, and text-to-SQL tasks, where they demonstrated strong performance, even surpassing larger models in some areas. The report concludes with suggestions for future research, such as scaling model size and enhancing reasoning abilities.
📎 Link to paperTue, 12 Nov 2024 - 45 - Attacking Vision-Language Computer Agents via Pop-ups
😈 Attacking Vision-Language Computer Agents via Pop-ups
This research paper examines vulnerabilities in vision-language models (VLMs) that power autonomous agents performing computer tasks. The authors show that these VLM agents can be easily tricked into clicking on carefully crafted malicious pop-ups, which humans would typically recognize and avoid. These deceptive pop-ups mislead the agents, disrupting their task performance and reducing success rates. The study tests various pop-up designs across different VLM agents and finds that even simple countermeasures, such as instructing the agent to ignore pop-ups, are ineffective. The authors conclude that these vulnerabilities highlight serious security risks and call for more robust safety measures to ensure reliable agent performance.
📎 Link to paperSat, 09 Nov 2024 - 44 - Number Cookbook
📓 Number Cookbook: Number Understanding of Language Models and How to Improve It
This research paper examines the numerical understanding and processing abilities (NUPA) of large language models (LLMs). The authors create a benchmark to test LLMs on four numerical representations (integers, floating-point numbers, fractions, and scientific notation) across 17 tasks grouped into four ability categories. They find that, despite strong problem-solving capabilities, LLMs struggle with basic numerical operations. The paper evaluates methods to enhance NUPA during pretraining and finetuning, such as specialized tokenizers, positional encodings, and data formats, and notes the limitations of chain-of-thought techniques for numerical tasks. The authors call for further research to improve LLMs' fundamental numerical capabilities.
📎 Link to paperFri, 08 Nov 2024 - 43 - Jigsaw Puzzles
🧩 Jigsaw Puzzles: Splitting Harmful Questions to Jailbreak Large Language Models
This research paper investigates the vulnerabilities of large language models (LLMs) to "jailbreak" attacks, where malicious users attempt to trick the model into generating harmful content. The authors propose a new attack strategy called Jigsaw Puzzles (JSP) which breaks down harmful questions into harmless fractions and feeds them to the LLM in multiple turns, bypassing the model's built-in safeguards. The paper explores the effectiveness of JSP across different LLM models and harmful categories, analyzing the role of various prompt designs and splitting strategies. The authors also compare JSP's performance to other existing jailbreak methods and demonstrate its ability to overcome various defense mechanisms. The paper concludes by highlighting the importance of continued research and development of more robust defenses against such attacks.
📎 Link to paperThu, 07 Nov 2024 - 42 - Multi-expert Prompting with LLMs
🤝 Multi-expert Prompting with LLMs
The research paper presents Multi-expert Prompting, a novel method for improving the reliability, safety, and usefulness of Large Language Models (LLMs). Multi-expert Prompting simulates multiple experts within an LLM, collecting their answers to an instruction and aggregating them into a final response. This process leverages the Nominal Group Technique, a human-designed decision-making framework, to ensure a balanced and comprehensive output, surpassing the limitations of single-expert approaches. The authors demonstrate the method’s effectiveness through thorough evaluation on various benchmarks, highlighting its significant improvements in truthfulness, factuality, toxicity reduction, and overall informativeness compared to existing baselines.
📎 Link to paperTue, 05 Nov 2024 - 41 - Investigating the Role of Prompting and External Tools in Hallucination Rates of LLMs
🔎 Investigating the Role of Prompting and External Tools in Hallucination Rates of Large Language Models
This paper examines the effectiveness of different prompting techniques and frameworks for mitigating hallucinations in large language models (LLMs). The authors investigate how these techniques, including Chain-of-Thought, Self-Consistency, and Multiagent Debate, can improve reasoning capabilities and reduce factual inconsistencies. They also explore the impact of LLM agents, which are AI systems designed to perform complex tasks by combining LLMs with external tools, on hallucination rates. The study finds that the best strategy for reducing hallucinations depends on the specific NLP task, and that while external tools can extend the capabilities of LLMs, they can also introduce new hallucinations.
📎 Link to paperSun, 03 Nov 2024 - 40 - Mind Your Step (by Step)
🌀 Mind Your Step (by Step): Chain-of-Thought can Reduce Performance on Tasks where Thinking Makes Humans Worse
This research paper examines how chain-of-thought (CoT) prompting—encouraging models to reason step-by-step—affects large language and multimodal model performance across tasks. While CoT generally boosts performance, the authors find it significantly hampers model accuracy in three specific contexts: implicit statistical learning, facial recognition, and classifying data with exceptions. The paper suggests a similarity between CoT and human verbal reasoning, proposing that tasks where deliberate thinking harms human performance may similarly impair models using CoT. The study concludes that recognizing scenarios where reasoning is counterproductive for humans can highlight situations where CoT also hinders model effectiveness.
📎 Link to paperSat, 02 Nov 2024 - 39 - SimpleQA
❓Measuring short-form factuality in large language models
This document introduces SimpleQA, a new benchmark for evaluating the factuality of large language models. The benchmark consists of over 4,000 short, fact-seeking questions designed to be challenging for advanced models, with a focus on ensuring a single, indisputable answer. The authors argue that SimpleQA is a valuable tool for assessing whether models "know what they know", meaning their ability to correctly answer questions with high confidence. They further explore the calibration of language models, investigating the correlation between confidence and accuracy, as well as the consistency of responses when the same question is posed multiple times. The authors conclude that SimpleQA provides a valuable framework for evaluating the factuality of language models and encourages the development of more trustworthy and reliable models.
📎 Link to paper
🌐 Read their blogThu, 31 Oct 2024 - 38 - GPT-4o System Card
📜 GPT-4o System Card
This technical document is the System Card for OpenAI's GPT-4o, a multimodal, autoregressive language model that can process and generate text, audio, images, and video. The card provides a detailed overview of the model's capabilities, limitations, and safety evaluations across various categories, with a particular focus on its speech-to-speech (voice) capabilities. The card details the model's training data, including web data, code and math, and multimodal data. It also covers OpenAI's risk identification, assessment, and mitigation strategies, including red teaming, evaluation methodologies, and observed safety challenges. The document examines the potential societal impacts of the model, including anthropomorphization and emotional reliance, health applications, and scientific capabilities. Finally, the card concludes with a discussion of the next steps for research and development in omni models.
📎 Link to paperWed, 30 Oct 2024 - 37 - Mixture of Parrots
🦜 Mixture of Parrots: Experts improve memorization more than reasoning
This research paper investigates the effectiveness of Mixture-of-Experts (MoE) architectures in deep learning, particularly comparing their performance to standard dense transformers. The authors demonstrate through theoretical analysis and empirical experiments that MoEs excel at memory-intensive tasks, leveraging a large number of experts to effectively memorize data. However, for reasoning-based tasks, they find MoEs offer limited performance gains compared to dense models, suggesting that scaling the dimension of the model is more beneficial in such scenarios. The study provides valuable insights into the strengths and weaknesses of MoE architectures, highlighting their potential as memory machines while emphasizing the need for alternative approaches for tasks demanding strong reasoning capabilities.
📎 Link to paperTue, 29 Oct 2024 - 36 - Improve Vision Language Model Chain-of-thought Reasoning
🖼 Improve Vision Language Model Chain-of-thought Reasoning
This research paper investigates how to improve the chain-of-thought (CoT) reasoning capabilities of vision language models (VLMs). The authors address the lack of high-quality CoT data for training VLMs and propose two key methods: first, distilling rationales from a powerful language model (GPT-4o) to enrich the training data and fine-tune VLMs, leading to significant improvements in CoT performance. Second, they leverage reinforcement learning (RL) through the Direct Preference Optimization (DPO) algorithm to further calibrate reasoning quality, utilizing positive and negative pairs of model-generated reasoning chains. The authors demonstrate that their approach effectively enhances reasoning capabilities, paving the way for more robust and interpretable multimodal models.
📎 Link to paperMon, 28 Oct 2024 - 35 - Breaking the Memory Barrier
🧠 Breaking the Memory Barrier: Near Infinite Batch Size Scaling for Contrastive Loss
This research paper introduces Inf-CL, a novel approach for contrastive learning that dramatically reduces GPU memory usage during training, allowing for near-infinite batch sizes. The authors address the issue of quadratic memory growth in traditional methods by implementing a tile-based computation strategy that partitions the contrastive loss calculation into smaller, sequentially computed blocks. To further enhance efficiency, they propose a multi-level tiling strategy that leverages ring-based communication at the GPU level and fused kernels at the CUDA core level, minimizing I/O overhead. The experiments demonstrate that Inf-CL significantly outperforms previous methods, achieving unprecedented batch sizes while maintaining accuracy and comparable training speed. This breakthrough opens new possibilities for large-scale contrastive learning, paving the way for advancements in areas such as self-supervised learning and dense text retrieval.
📎 Link to paperSun, 27 Oct 2024 - 34 - LLMs Reflect the Ideology of their Creators
⚖️ Large Language Models Reflect the Ideology of their Creators
This study examines the ideological stances of large language models (LLMs) by analyzing their responses to prompts about a vast set of historical figures. The authors discovered that LLMs often reflect the worldview of their creators, demonstrating significant differences in their evaluations of political figures depending on the prompting language, the region of their creation, and even the company that developed them. The study reveals that LLMs are not ideologically neutral and raises concerns about the potential for political manipulation and the need for transparency and regulation in the development and use of LLMs.
📎 Link to paperSat, 26 Oct 2024 - 33 - LongRAG
📜 LongRAG: A Dual-Perspective Retrieval-Augmented Generation Paradigm for Long-Context Question Answering
The source is a research paper that proposes a new approach called LongRAG for enhancing the performance of Retrieval-Augmented Generation (RAG) systems in Long-Context Question Answering (LCQA) tasks. LongRAG addresses two major issues that limit the effectiveness of traditional RAG systems: the "lost in the middle" problem, where relevant information within long contexts is often missed, and the challenge of identifying precise factual details amid noise. This new paradigm uses a dual-perspective approach that effectively integrates global long-context information with specific factual details. The researchers demonstrate that LongRAG significantly outperforms other LCQA methods and traditional RAG systems, including those using large language models, on three multi-hop datasets.
📎 Link to paperFri, 25 Oct 2024 - 32 - A Theoretical Understanding of Chain-of-Thought
⛓️ A Theoretical Understanding of Chain-of-Thought: Coherent Reasoning and Error-Aware Demonstration
The paper explores Chain-of-Thought (CoT) prompting, a method to enhance the reasoning skills of large language models (LLMs). It introduces Coherent CoT, where reasoning from previous steps is integrated during predictions, leading to better error correction and accuracy compared to a step-by-step approach. The study shows that errors in intermediate reasoning steps have a more significant impact on the final outcome than mistakes in the final response. Based on this, the authors propose an error-aware CoT prompting method, which includes both correct and incorrect reasoning in demonstrations, allowing LLMs to improve reasoning by learning from earlier mistakes.
🔗 Link to paperThu, 24 Oct 2024 - 31 - A Survey on Data Synthesis and Augmentation for Large Language Models
📚 A Survey on Data Synthesis and Augmentation for Large Language Models
This research paper examines the use of synthetic and augmented data to enhance the capabilities of Large Language Models (LLMs). The authors argue that the rapid growth of LLMs is outpacing the availability of high-quality data, creating a data exhaustion crisis. To address this challenge, the paper analyzes different data generation methods, including data augmentation and data synthesis, and explores their applications throughout the lifecycle of LLMs, including data preparation, pre-training, fine-tuning, instruction-tuning, and preference alignment. The paper also discusses the challenges associated with these techniques, such as data quality and bias, and proposes future research directions for the field.
📎 Link to paperWed, 23 Oct 2024 - 30 - Revealing the Barriers of Language Agents in Planning
🤔 Revealing the Barriers of Language Agents in Planning
This research paper examines the challenges faced by language agents in planning tasks. The authors explore the reasons behind the shortcomings of these agents, particularly their limited understanding of constraints and their diminishing ability to focus on goals as the planning horizon lengthens. They investigate two common strategies for improving planning performance: episodic memory updating and parametric memory updating. The study concludes that these strategies, while offering some improvements, primarily function as shortcut learning mechanisms, falling short of achieving human-level planning abilities.
📎 Link to paperTue, 22 Oct 2024 - 29 - Intelligence at the Edge of Chaos
🔀 Intelligence at the Edge of Chaos
This research investigates how intelligent behavior emerges in artificial systems by studying the connection between the complexity of rule-based systems and the abilities of models trained to predict these rules. The researchers used elementary cellular automata (ECA), simple one-dimensional systems with varying complexity, to train large language models (LLMs). Their results show that models trained on more complex ECAs demonstrate greater intelligence, excelling in reasoning and chess move prediction tasks. A key finding is the importance of training at a "sweet spot" of complexity—known as the "edge of chaos"—where systems are structured yet difficult to predict, fostering intelligent behavior. Additionally, models trained on complex rules develop sophisticated solutions by incorporating information from previous states, which improves their ability to generalize and perform well on various tasks.
📎 Link to paperMon, 21 Oct 2024 - 28 - Inference Scaling for Long-Context RAG
🗓 Inference Scaling for Long-Context Retrieval Augmented Generation
This research paper explores the effectiveness of inference scaling for retrieval augmented generation (RAG), a technique that enhances large language models (LLMs) by incorporating external knowledge. The authors introduce two strategies, demonstration-based RAG (DRAG) and iterative demonstration-based RAG (IterDRAG), for effectively scaling inference computation. They demonstrate that increasing inference computation, when optimally allocated, leads to nearly linear gains in RAG performance. Furthermore, they develop a computation allocation model to predict the optimal test-time compute allocation for various tasks and scenarios, showcasing its effectiveness in achieving performance gains and aligning with experimental results.
📎 Link to paperSun, 20 Oct 2024 - 27 - Model Swarms
🤝 Model Swarms: Collaborative Search to Adapt LLM Experts via Swarm Intelligence
This paper presents a new method called MODEL SWARMS, a collaborative search algorithm for adapting large language models (LLMs) using swarm intelligence. The researchers propose viewing each LLM expert as a "particle" in a swarm and use particle swarm optimization (PSO) to collaboratively search the weight space for optimized models. This approach allows LLMs to adapt to a variety of objectives, including single tasks, multi-task domains, reward models, and human interests, without requiring large amounts of training data. Extensive experiments demonstrate that MODEL SWARMS outperforms existing model composition baselines and enables the discovery of previously unseen capabilities in LLMs.
📎 Link to paperSat, 19 Oct 2024 - 26 - Agent-as-a-Judge
🤖 Agent-as-a-Judge: Evaluate Agents with Agents
The paper detail a new framework for evaluating agentic systems called Agent-as-a-Judge, which uses other agentic systems to assess their performance. To test this framework, the authors created DevAI, a benchmark dataset consisting of 55 realistic automated AI development tasks. They compared Agent-as-a-Judge to LLM-as-a-Judge and Human-as-a-Judge on DevAI, finding that Agent-as-a-Judge outperforms both, aligning closely with human evaluations. The authors also discuss the benefits of Agent-as-a-Judge for providing intermediate feedback and creating a flywheel effect, where both the judge and evaluated agents improve through an iterative process.
📎 Link to paper
🤗 See their HuggingFaceFri, 18 Oct 2024 - 25 - First-Person Fairness in Chatbots
⚖️ First-Person Fairness in Chatbots
This paper from OpenAI examines potential bias in chatbot systems like ChatGPT, specifically focusing on how a user's name, which can be associated with demographic attributes, influences the chatbot's responses. The authors propose a privacy-preserving method to measure user name bias across a large dataset of real-world chatbot interactions. They identify several instances of bias, demonstrating that chatbot responses can show a tendency towards creating protagonists whose gender matches the user's likely gender and that users with female-associated names receive responses with friendlier and simpler language more often. The study also finds that post-training interventions like reinforcement learning can significantly mitigate harmful stereotypes.
📎 Link to paper
🌐 Read their blogFri, 18 Oct 2024 - 24 - Thinking LLMs
🤔 Thinking LLMs: General Instruction Following with Thought Generation
This research paper explores the concept of "Thinking LLMs," or large language models that can generate internal thoughts before responding to user prompts. The authors propose a training method called Thought Preference Optimization (TPO) which uses an iterative process to encourage LLMs to develop thinking abilities. TPO leverages an existing judge model that evaluates responses, implicitly guiding the model to improve its thoughts based on the quality of the resulting responses. The study demonstrates that Thinking LLMs can outperform standard LLMs on various general instruction-following tasks, including those not typically associated with reasoning, such as marketing and health. The research highlights the potential for Thinking LLMs to expand the capabilities of these models beyond traditional reasoning and problem-solving domains.
📎 Link to paperFri, 18 Oct 2024 - 23 - Addition is All You Need
🔋 Addition is All You Need for Energy-efficient Language Models
This research paper introduces a novel algorithm called Linear-Complexity Multiplication (L-Mul) that aims to make language models more energy-efficient. L-Mul replaces computationally expensive floating-point multiplications with integer addition operations, significantly reducing energy consumption. The authors demonstrate that L-Mul achieves high precision, even surpassing 8-bit floating-point multiplications in certain cases. They evaluate L-Mul on various benchmarks, including natural language, vision, and mathematics tasks, showing that L-Mul can be effectively implemented in attention mechanisms without compromising performance, leading to significant energy savings in model deployment. The authors conclude that L-Mul holds great potential for creating more energy-efficient and cost-effective AI systems.
📎 Link to paperFri, 18 Oct 2024 - 22 - MLE-bench
🤖 MLE-bench: Evaluating Machine Learning Agents on Machine Learning Engineering
The paper introduces MLE-bench, a benchmark designed to evaluate AI agents' ability to perform machine learning engineering tasks. The benchmark comprises 75 Kaggle competitions, each requiring agents to solve real-world problems involving data preparation, model training, and code debugging. Researchers evaluated several cutting-edge language models on MLE-bench, with the best-performing setup achieving at least a bronze medal in 16.9% of the competitions. The paper investigates various factors influencing performance, such as resource scaling and contamination from pre-training, and concludes that while current agents demonstrate promising capabilities, significant challenges remain.
📎 Link to paperFri, 18 Oct 2024 - 21 - Long-Context LLMs Meet RAG
📈 Long-Context LLMs Meet RAG: Overcoming Challenges for Long Inputs in RAG
This paper explores the challenges and opportunities of using long-context language models (LLMs) in retrieval-augmented generation (RAG) systems. While increasing the number of retrieved passages initially improves performance, the authors find that it eventually degrades due to the introduction of irrelevant information, or "hard negatives." To address this, the paper proposes three methods for enhancing the robustness of RAG with long-context LLMs: retrieval reordering, RAG-specific implicit LLM fine-tuning, and RAG-oriented LLM fine-tuning with intermediate reasoning. The paper also investigates the impact of various factors related to data distribution, retriever selection, and training context length on the effectiveness of RAG-specific tuning.
📎 Link to paperFri, 18 Oct 2024 - 20 - GSM-Symbolic
📊 GSM-Symbolic: Understanding the Limitations of Mathematical Reasoning in Large Language Models
The paper investigates the mathematical reasoning abilities of large language models (LLMs). The authors created a new benchmark, GSM-Symbolic, to test LLMs' performance in a more reliable way. The results show that LLMs perform poorly and inconsistently across different versions of the same question, indicating a fragility in their reasoning abilities. Additionally, the models are sensitive to irrelevant information, suggesting they may be relying on pattern matching rather than true logical reasoning. The study concludes that LLMs still have significant limitations in performing genuine mathematical reasoning and emphasizes the need for further research to develop more robust and logical models.
📎 Link to paperFri, 18 Oct 2024 - 19 - Anti-Social LLM
😶 Anti-Social Behavior and Persuasion Ability of LLMs
This study explores the behavior of Large Language Models (LLMs) in a simulated prison environment, inspired by the Stanford Prison Experiment. It focuses on two key aspects: persuasion, where a prisoner tries to convince a guard to grant more yard time or help escape, and anti-social behavior, such as toxicity and violence. The analysis reveals that some models, like Mixtral and Mistral, struggle to maintain their assigned roles. Persuasion is more successful when asking for yard time than for escape. Additionally, the guard's personality significantly affects the occurrence of anti-social behavior, while the prisoner's goal has minimal impact. The study underscores the importance of addressing potential negative behaviors in AI interactions, emphasizing the need for safeguards and more research on AI safety and ethics.
📎 Link to paperFri, 18 Oct 2024 - 18 - Differential Transformer
🎧 Differential Transformer
The paper introduces the Differential Transformer, a new architecture for large language models (LLMs) that aims to improve their ability to focus on relevant information within long sequences. It achieves this by introducing a differential attention mechanism which calculates attention scores as the difference between two separate softmax attention maps, effectively canceling out noise and promoting sparse attention patterns. This enhanced focus on relevant context leads to improvements in various tasks, including long-context modeling, key information retrieval, hallucination mitigation, in-context learning, and reducing activation outliers. The paper provides experimental evidence to support these claims, showcasing the Differential Transformer's superiority over traditional Transformers in several scenarios.
📎 Link to paperFri, 18 Oct 2024 - 17 - ToolGen
🛠 ToolGen: Unified Tool Retrieval and Calling via Generation
This research paper introduces ToolGen, a novel framework that enables LLMs to directly access and utilize external tools by representing each tool as a unique token within the model's vocabulary. ToolGen addresses the limitations of traditional tool retrieval methods, which often rely on separate retrieval mechanisms and are constrained by context length. The paper describes a three-stage training process for ToolGen, consisting of tool memorization, retrieval training, and end-to-end agent tuning, which allows LLMs to learn and utilize a vast number of tools effectively and efficiently. Experimental results demonstrate that ToolGen outperforms existing approaches in both tool retrieval and autonomous task completion, highlighting its potential to revolutionize AI agent capabilities.
📎 Link to paper
🌐 Check their GithubFri, 18 Oct 2024 - 16 - LangGPT
👨🔧 Minstrel: Structural Prompt Generation with Multi-Agents Coordination for Non-AI Experts
This research proposes LangGPT, a structural prompt framework for designing prompts to instruct Large Language Models (LLMs). This framework, inspired by programming languages, provides a systematic and reusable method for creating prompts that are easier for non-AI experts to understand and use. The authors also present Minstrel, a multi-agent system that automatically generates these structural prompts, further reducing the learning cost and complexity of prompt design. The study demonstrates that using LangGPT prompts, either manually designed or generated by Minstrel, can significantly enhance the performance of LLMs compared to traditional prompt methods. The research also explores the ease of use and user satisfaction of LangGPT through a survey conducted within their online community.
📎 Link to paper
🌐See their GithubFri, 18 Oct 2024 - 15 - Movie Gen
🎞 Movie Gen: A Cast of Media Foundation Models
Meta AI researchers have introduced Movie Gen, a suite of foundation models capable of generating high-quality video and audio. Movie Gen models can synthesize videos based on text prompts, personalize videos using a user’s image, precisely edit videos with text instructions, and generate synchronized audio for videos. The research paper details the models' architecture, training procedures, and evaluation results, demonstrating their superior performance compared to existing methods in each of these areas. The paper also explores various aspects of model scaling, including parallelism techniques and data curation strategies used to train these large-scale media generation models.
📎 Link to paper
🌐 Read their blogFri, 18 Oct 2024 - 14 - LLMs Know More Than They Show
🕵️♀️ LLMs Know More Than They Show
This research examines the inner workings of large language models (LLMs) to understand and reduce their tendency to generate false information, known as "hallucinations." The authors find that LLMs internally encode information about the truthfulness of their outputs, with these signals concentrated in tokens related to exact answers. However, these truth signals are task-specific and may not apply universally across different tasks. They also find that LLMs' internal representations can predict error types, enabling more targeted error mitigation strategies. Interestingly, LLMs sometimes internally recognize the correct answer but still produce an incorrect one, highlighting a disconnect between internal knowledge and external output. This suggests potential for using LLMs' internal knowledge to reduce errors, requiring further study.
📎 Link to paperFri, 18 Oct 2024 - 13 - Were RNNs All We Needed?
🔁 Were RNNs All We Needed?
The paper "Were RNNs All We Needed?" examines the efficiency of traditional recurrent neural networks (RNNs), specifically LSTMs and GRUs, for long sequences. The authors demonstrate that by removing hidden state dependencies from their input, forget, and update gates, LSTMs and GRUs can be trained efficiently using the parallel prefix scan algorithm, resulting in significantly faster training times. They introduce simplified versions of these RNNs, called minLSTMs and minGRUs, which use fewer parameters and achieve performance comparable to recent sequence models like Transformers and Mamba. The paper highlights the potential for RNNs to be competitive alternatives to Transformers, particularly for long sequences, and raises the question of whether RNNs were all that was needed for sequence modeling.
📎 Link to paperFri, 18 Oct 2024 - 12 - SLMs, A Survey
📱 Small Language Models: Survey, Measurements, and Insights
This research paper reviews small language models (SLMs), which are optimized for use on devices with limited resources, such as smartphones. It covers recent advancements in SLM architectures, training datasets, and algorithms, and benchmarks their performance on tasks like commonsense reasoning, problem-solving, and mathematics. The paper also assesses the models' runtime efficiency on various hardware platforms and the effect of quantization techniques on performance. The authors highlight future research areas, including co-designing SLM architectures with device processors, creating high-quality synthetic datasets, and developing scaling laws that account for deployment constraints.
📎 Link to paperFri, 18 Oct 2024 - 11 - o1 in Medicine
💊 A Preliminary Study of o1 in Medicine
The research paper focuses on the performance of a new large language model (LLM) called o1 in the medical domain. o1 was trained with an internalized chain-of-thought technique using reinforcement learning strategies, which enhances its reasoning abilities. The paper evaluates o1 across three key aspects: understanding, reasoning, and multilinguality, using a diverse range of medical datasets. The researchers found that o1 demonstrates improved understanding and reasoning abilities compared to other LLMs, including GPT-4, and surpasses its predecessor in accuracy across a variety of tasks. However, o1 still struggles with hallucination, inconsistent multilingual ability, and biased evaluation metrics, which highlights the need for further research in these areas.
📎 Link to paperFri, 18 Oct 2024 - 10 - RAG and Beyond
📑 RAG and Beyond
This paper provides a comprehensive survey of the current state of data-augmented Large Language Models (LLMs), focusing on Retrieval-Augmented Generation (RAG) and beyond. The authors classify different types of queries that utilize external data into four levels based on their complexity: explicit fact queries, implicit fact queries, interpretable rationale queries, and hidden rationale queries. They discuss the specific challenges associated with each level and provide a detailed overview of the most effective techniques for addressing them, such as RAG, prompt tuning, in-context learning, and fine-tuning. The paper ultimately aims to guide developers in systematically developing data-augmented LLM applications by offering solutions to the various challenges faced at each query level.
📎 Link to paperFri, 18 Oct 2024 - 9 - Molmo and PixMo
🔓 Molmo and PixMo: Open Weights and Open Data for State-of-the-Art Multimodal Models
This research paper introduces Molmo, a new family of vision-language models (VLMs) that surpasses existing open-weight models in performance while maintaining open weights, data, and code. The key innovation is the collection of a large, detailed image caption dataset using speech-based descriptions, avoiding reliance on synthetic data generated by proprietary VLMs. Molmo is trained on this dataset, along with a diverse mixture of fine-tuning datasets, to achieve state-of-the-art performance on multiple academic benchmarks and human evaluation, even compared to proprietary systems like GPT-4o. The paper emphasizes the importance of open research and provides a comprehensive overview of the model architecture, data collection methods, training process, and evaluation results.
📎 Link to paper
🟣 Try their demoFri, 18 Oct 2024 - 8 - Self-Taught Evaluators
🔄 Self-Taught Evaluators
This research paper explores the development of self-taught language model evaluators. Instead of relying on costly human annotations, this approach utilizes synthetic data generated by the model itself. The method iteratively trains an LLM-as-a-Judge by creating contrasting response pairs, generating reasoning traces, and fine-tuning the model on this synthetic data. The research demonstrates that this method significantly improves the accuracy of the evaluator on benchmarks like RewardBench, achieving performance comparable to reward models trained with labeled examples. The authors also explore various data sources, ablations, and analyses to understand the effectiveness of the proposed approach.
📎 Link to paper
🌐 Link to their tweetFri, 18 Oct 2024 - 7 - Larger LLMs Become Less Reliable
⚠️ Larger and more instructable language models become less reliable
This research paper from Nature explores the relationship between the size and instructability of large language models (LLMs) and their reliability. The study finds that while larger, more instructable LLMs tend to perform better on complex tasks, they become less reliable in handling simple tasks, often producing plausible but incorrect answers instead of safely avoiding them. Additionally, the study highlights the limitations of human supervision in correcting errors and emphasizes the need for a fundamental shift in LLM design and development to prioritize reliability, particularly in high-stakes applications where predictable error distributions are crucial.
📎 Link to paperFri, 18 Oct 2024 - 6 - Logic-of-Thought
💭 Logic-of-Thought: Injecting Logic into Contexts for Full Reasoning in LLMs
This research paper introduces Logic-of-Thought (LoT), a novel prompting method designed to enhance logical reasoning in large language models. LoT extracts propositions and logical relations from input text, expands them using logical rules, and reintegrates this information into the original prompt. Unlike existing techniques, LoT preserves information and guides the model's reasoning process while leveraging its natural language understanding. Experiments across multiple datasets demonstrate LoT's effectiveness in improving various prompting methods. The authors also compare LoT favorably to a neuro-symbolic approach, highlighting its advantages in information preservation and language comprehension utilization.
📎 Link to paperFri, 18 Oct 2024 - 5 - Moshi
🟢 Moshi: a speech-text foundation model for real-time dialogue
The paper discusses a new multimodal foundation model called Moshi designed for real-time, full-duplex spoken dialogue. This model uses a text-based LLM called Helium to provide reasoning abilities and a neural audio codec called Mimi to encode audio into tokens. Moshi is innovative because it can handle overlapping speech and model both the user's and the system's speech in a single stream. The paper also explores the model's performance on various tasks like question answering and its ability to generate speech in different voices. Finally, it addresses safety concerns such as toxicity, regurgitation, and voice consistency, and proposes solutions using watermarking techniques.
📎 Link to paper
🤖 Try their demoFri, 18 Oct 2024 - 4 - Jailbreaking Large Language Models with Symbolic Mathematics
🔑 Jailbreaking Large Language Models with Symbolic Mathematics
This research paper investigates a new vulnerability in AI safety mechanisms by introducing MathPrompt, a technique that utilizes symbolic mathematics to bypass LLM safety measures. The paper demonstrates that encoding harmful natural language prompts into mathematical problems allows LLMs to generate harmful content, despite being trained to prevent it. Experiments across 13 state-of-the-art LLMs show a high success rate for MathPrompt, indicating that existing safety measures are not effective against mathematically encoded inputs. The study emphasizes the need for more comprehensive safety mechanisms that can handle various input types and their associated risks.
📎 Link to paperFri, 18 Oct 2024 - 3 - LLMs Still Can't Plan; Can LRMs?
📈 LLMs Still Can't Plan; Can LRMs?
The paper "LLMs Still Can't Plan; Can LRMs? A Preliminary Evaluation of OpenAI's o1 on PlanBench" investigates the ability of large language models (LLMs) to plan, using a benchmark called PlanBench. The authors find that while OpenAI's new "Large Reasoning Model" (LRM) o1 shows significant improvement in planning abilities, it still falls short of fully achieving the task. This research highlights the need for further investigation into the accuracy, efficiency, and guarantees associated with these advanced models.
📎 Link to paperFri, 18 Oct 2024 - 2 - A Comprehensive Evaluation of Quantized Instruction-Tuned LLMs
📏 A Comprehensive Evaluation of Quantized Instruction-Tuned LLMs
This paper, titled "A Comprehensive Evaluation of Quantized Instruction-Tuned Large Language Models: An Experimental Analysis up to 405B," examines the performance of large language models (LLMs) after they have been compressed using various quantization methods. The authors assess the impact of these techniques on different task types and model sizes, including the very large 405B parameter Llama 3.1 model. They explore how different quantization methods, model sizes, and bit-widths affect performance, finding that larger quantized models often outperform smaller FP16 models and that certain methods, such as weight-only quantization, are particularly effective for larger models. The study also concludes that task difficulty does not significantly impact the accuracy degradation caused by quantization.
📎 Link to paperFri, 18 Oct 2024 - 1 - On the Diagram of Thought
🧠 On the Diagram of Thought
This paper introduces a new framework called Diagram of Thought (DoT) that models how large language models (LLMs) reason. Unlike traditional methods that represent reasoning as linear chains or trees, DoT utilizes a directed acyclic graph (DAG) structure. This structure allows LLMs to navigate complex reasoning pathways while ensuring logical consistency. By incorporating feedback mechanisms and leveraging auto-regressive next-token prediction, DoT enables LLMs to iteratively refine their reasoning process. The authors also formalize the DoT framework using Topos Theory, providing a mathematical foundation for its logical consistency and soundness. This approach enhances both training and inference within a single LLM, eliminating the need for multiple models or external control mechanisms. DoT offers a promising framework for developing next-generation reasoning-specialized LLMs.
📎 Link to paperThu, 17 Oct 2024
Podcasts similares a LlamaCast
Global News Podcast BBC World Service
Kriminálka Český rozhlas
El Partidazo de COPE COPE
Herrera en COPE COPE
The Dan Bongino Show Cumulus Podcast Network | Dan Bongino
Es la Mañana de Federico esRadio
La Noche de Dieter esRadio
Hondelatte Raconte - Christophe Hondelatte Europe 1
Affaires sensibles France Inter
La rosa de los vientos OndaCero
Más de uno OndaCero
La Zanzara Radio 24
Espacio en blanco Radio Nacional
Les Grosses Têtes RTL
L'Heure Du Crime RTL
El Larguero SER Podcast
Nadie Sabe Nada SER Podcast
SER Historia SER Podcast
Todo Concostrina SER Podcast
安住紳一郎の日曜天国 TBS RADIO
The Tucker Carlson Show Tucker Carlson Network
辛坊治郎 ズーム そこまで言うか! ニッポン放送
飯田浩司のOK! Cozy up! Podcast ニッポン放送
武田鉄矢・今朝の三枚おろし 文化放送PodcastQR