Filtra per genere
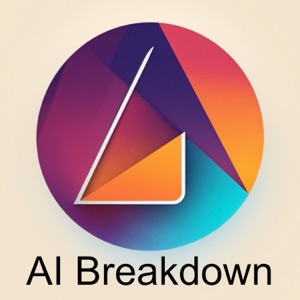
The podcast where we use AI to breakdown the recent AI papers and provide simplified explanations of intricate AI topics for educational purposes. The content presented here is generated automatically by utilizing LLM and text to speech technologies. While every effort is made to ensure accuracy, any potential misrepresentations or inaccuracies are unintentional due to evolving technology. We value your feedback to enhance our podcast and provide you with the best possible learning experience.
- 572 - Arxiv Paper - Qwen2-VL: Enhancing Vision-Language Model’s Perception of the World at Any Resolution
In this episode, we discuss Qwen2-VL: Enhancing Vision-Language Model's Perception of the World at Any Resolution by Peng Wang, Shuai Bai, Sinan Tan, Shijie Wang, Zhihao Fan, Jinze Bai, Keqin Chen, Xuejing Liu, Jialin Wang, Wenbin Ge, Yang Fan, Kai Dang, Mengfei Du, Xuancheng Ren, Rui Men, Dayiheng Liu, Chang Zhou, Jingren Zhou, Junyang Lin. The Qwen2-VL Series introduces Naive Dynamic Resolution for processing images of varying resolutions more efficiently and integrates Multimodal Rotary Position Embedding for improved fusion of positional information across modalities. It employs a unified approach for both images and videos, enhancing visual perception and explores scaling laws for large vision-language models by increasing model size and training data. The Qwen2-VL-72B model achieves competitive performance, rivaling top models like GPT-4o and Claude3.5-Sonnet, and surpasses other generalist models across various benchmarks.
Thu, 14 Nov 2024 - 04min - 571 - Arxiv Paper - FasterCache: Training-Free Video Diffusion Model Acceleration with High Quality
In this episode, we discuss FasterCache: Training-Free Video Diffusion Model Acceleration with High Quality by Zhengyao Lv, Chenyang Si, Junhao Song, Zhenyu Yang, Yu Qiao, Ziwei Liu, Kwan-Yee K. Wong. FasterCache is introduced as a training-free approach that accelerates inference in video diffusion models by reusing features more efficiently, maintaining high video quality. The strategy involves a dynamic feature reuse method and CFG-Cache, which enhances the reuse of conditional and unconditional outputs, effectively reducing redundancy without loss of subtle variations. Experimental results demonstrate that FasterCache offers significant speed improvements, such as a 1.67× increase on Vchitect-2.0, while preserving video quality, outperforming previous acceleration methods.
Tue, 12 Nov 2024 - 04min - 570 - Arxiv Paper - Relaxed Recursive Transformers: Effective Parameter Sharing with Layer-wise LoRA
In this episode, we discuss Relaxed Recursive Transformers: Effective Parameter Sharing with Layer-wise LoRA by Sangmin Bae, Adam Fisch, Hrayr Harutyunyan, Ziwei Ji, Seungyeon Kim, Tal Schuster. The paper presents methods to transform large language models into smaller, efficient "Recursive Transformers" by using parameter sharing through revisiting "layer tying", which reduces model size and cost with minimal performance loss. By initializing these Recursive Transformers from standard pre-trained models and incorporating "Relaxed Recursive Transformers" with LoRA modules for flexibility, the models can recover most of the original performance while remaining compact. Additionally, a new inference paradigm called Continuous Depth-wise Batching with early exiting is introduced, aiming to enhance inference throughput significantly.
Mon, 11 Nov 2024 - 04min - 569 - Arxiv Paper - Long Context RAG Performance of Large Language Models
In this episode, we discuss Long Context RAG Performance of Large Language Models by Quinn Leng, Jacob Portes, Sam Havens, Matei Zaharia, Michael Carbin. The paper examines the effects of long context lengths on Retrieval Augmented Generation (RAG) in large language models, especially with models supporting contexts over 64k tokens like Anthropic Claude and GPT-4-turbo. Experiments across 20 LLMs and varying context lengths revealed that only the advanced models maintain accuracy beyond this threshold. Additionally, the study highlights limitations and failure modes in RAG with extended context lengths, suggesting areas for future research.
Fri, 08 Nov 2024 - 03min - 568 - Arxiv Paper - NVLM: Open Frontier-Class Multimodal LLMs
In this episode, we discuss NVLM: Open Frontier-Class Multimodal LLMs by Wenliang Dai, Nayeon Lee, Boxin Wang, Zhuolin Yang, Zihan Liu, Jon Barker, Tuomas Rintamaki, Mohammad Shoeybi, Bryan Catanzaro, Wei Ping. The paper introduces NVLM 1.0, a set of advanced multimodal large language models that achieve state-of-the-art performance on vision-language tasks and improve upon their text-only capabilities. It outlines the benefits of a novel architecture that enhances training efficiency and reasoning abilities using a 1-D tile-tagging design, emphasizing the importance of dataset quality and task diversity over scale. NVLM 1.0's models excel in multimodal and text-only tasks through the integration of high-quality data, and the model weights are released with plans to open-source the training code.
Mon, 04 Nov 2024 - 04min - 567 - Arxiv Paper - ColPali: Efficient Document Retrieval with Vision Language Models
In this episode, we discuss ColPali: Efficient Document Retrieval with Vision Language Models by Manuel Faysse, Hugues Sibille, Tony Wu, Bilel Omrani, Gautier Viaud, Céline Hudelot, Pierre Colombo. The paper discusses the limitations of modern document retrieval systems in effectively utilizing visual elements, prompting the introduction of the Visual Document Retrieval Benchmark (ViDoRe) to evaluate systems on tasks involving rich visual content. To address these challenges, a new model architecture, ColPali, is proposed, which utilizes Vision Language Models to generate high-quality, context-aware embeddings from document page images. ColPali employs a late interaction matching mechanism, achieving superior performance over existing systems and offering faster, trainable-from-scratch solutions, with all project materials available online.
Fri, 01 Nov 2024 - 03min - 566 - Arxiv Paper - Molmo and PixMo: Open Weights and Open Data for State-of-the-Art Multimodal Models
In this episode, we discuss Molmo and PixMo: Open Weights and Open Data for State-of-the-Art Multimodal Models by Matt Deitke, Christopher Clark, Sangho Lee, Rohun Tripathi, Yue Yang, Jae Sung Park, Mohammadreza Salehi, Niklas Muennighoff, Kyle Lo, Luca Soldaini, Jiasen Lu, Taira Anderson, Erin Bransom, Kiana Ehsani, Huong Ngo, YenSung Chen, Ajay Patel, Mark Yatskar, Chris Callison-Burch, Andrew Head, Rose Hendrix, Favyen Bastani, Eli VanderBilt, Nathan Lambert, Yvonne Chou, Arnavi Chheda, Jenna Sparks, Sam Skjonsberg, Michael Schmitz, Aaron Sarnat, Byron Bischoff, Pete Walsh, Chris Newell, Piper Wolters, Tanmay Gupta, Kuo-Hao Zeng, Jon Borchardt, Dirk Groeneveld, Jen Dumas, Crystal Nam, Sophie Lebrecht, Caitlin Wittlif, Carissa Schoenick, Oscar Michel, Ranjay Krishna, Luca Weihs, Noah A. Smith, Hannaneh Hajishirzi, Ross Girshick, Ali Farhadi, Aniruddha Kembhavi. The paper presents Molmo, a new family of open visual language models (VLMs) designed to foster transparency and accessibility. Molmo's development includes a unique image caption dataset created using human speech-based descriptions and a mixed dataset for fine-tuning, incorporating Q&A and 2D pointing data. The 72B Molmo model surpasses both open-source and proprietary systems in performance, with plans to release all model weights, data, and source code.
Thu, 31 Oct 2024 - 04min - 565 - Arxiv Paper - Scaling Smart: Accelerating Large Language Model Pre-training with Small Model Initialization
In this episode, we discuss Scaling Smart: Accelerating Large Language Model Pre-training with Small Model Initialization by Mohammad Samragh, Iman Mirzadeh, Keivan Alizadeh Vahid, Fartash Faghri, Minsik Cho, Moin Nabi, Devang Naik, Mehrdad Farajtabar. The paper presents HyperCloning, a technique for initializing large language models with smaller, pre-trained models to leverage their predictive power. This method allows large models to require less training time and fewer GPU hours by scaling up small models while preserving their functionalities. HyperCloning offers a viable solution to efficiently manage the high costs and time investments in training large language models.
Wed, 30 Oct 2024 - 04min - 564 - Arxiv Paper - Unbounded: A Generative Infinite Game of Character Life Simulation
In this episode, we discuss Unbounded: A Generative Infinite Game of Character Life Simulation by Jialu Li, Yuanzhen Li, Neal Wadhwa, Yael Pritch, David E. Jacobs, Michael Rubinstein, Mohit Bansal, Nataniel Ruiz. The paper introduces UNBOUNDED, a generative infinite game utilizing generative AI models to create an open-ended, character life simulation game inspired by sandbox simulations. It presents innovations in AI, such as a specialized LLM for real-time generation of game mechanics and narratives, and an IP-Adapter for visually consistent character representation. The system is evaluated and shown to improve upon traditional methods in aspects such as character simulation, narrative coherence, and visual consistency.
Tue, 29 Oct 2024 - 04min - 563 - Arxiv Paper - Reverse Question Answering: Can an LLM Write a Question so Hard (or Bad) that it Can’t Answer?
In this episode, we discuss Reverse Question Answering: Can an LLM Write a Question so Hard (or Bad) that it Can't Answer? by Nishant Balepur, Feng Gu, Abhilasha Ravichander, Shi Feng, Jordan Boyd-Graber, Rachel Rudinger. The paper investigates the reverse question answering (RQA) task where a question is generated based on a given answer and examines how 16 large language models (LLMs) perform on this task compared to traditional question answering (QA). The study reveals that LLMs are less accurate in RQA for numerical answers but perform better with textual ones, and they often can answer their incorrectly generated questions accurately in traditional QA, indicating that errors are not solely due to knowledge gaps. Findings also highlight that RQA errors correlate with question difficulty and are inversely related to the frequency of answers in the data corpus, presenting challenges in generating valid multi-hop questions and suggesting areas for improvement in LLM reasoning for RQA.
Mon, 28 Oct 2024 - 04min - 562 - Arxiv Paper - LongVU: Spatiotemporal Adaptive Compression for Long Video-Language Understanding
In this episode, we discuss LongVU: Spatiotemporal Adaptive Compression for Long Video-Language Understanding by Xiaoqian Shen, Yunyang Xiong, Changsheng Zhao, Lemeng Wu, Jun Chen, Chenchen Zhu, Zechun Liu, Fanyi Xiao, Balakrishnan Varadarajan, Florian Bordes, Zhuang Liu, Hu Xu, Hyunwoo J. Kim, Bilge Soran, Raghuraman Krishnamoorthi, Mohamed Elhoseiny, Vikas Chandra. LongVU presents a spatiotemporal adaptive compression method for processing long videos using Multimodal Large Language Models, efficiently reducing redundancy while preserving important visual information. It employs techniques like cross-modal queries, DINOv2 features, and token reduction to manage spatial and temporal information. This approach shows superior performance on video understanding benchmarks, handling lengthy videos effectively and demonstrating scalability even in smaller models.
Thu, 24 Oct 2024 - 05min - 561 - Arxiv Paper - When Does Perceptual Alignment Benefit Vision Representations?
In this episode, we discuss When Does Perceptual Alignment Benefit Vision Representations? by Shobhita Sundaram, Stephanie Fu, Lukas Muttenthaler, Netanel Y. Tamir, Lucy Chai, Simon Kornblith, Trevor Darrell, Phillip Isola. The paper examines how aligning vision model representations with human perception affects various computer vision tasks by finetuning models on human similarity judgments and testing on standard benchmarks. The results show improved performance in tasks such as counting, segmentation, and retrieval, without negatively impacting performance in specialized domains like medical imaging. The study suggests that integrating human perceptual bias into vision models can enhance their representation capabilities.
Wed, 23 Oct 2024 - 04min - 560 - Arxiv paper - SceneCraft: Layout-Guided 3D Scene Generation
In this episode, we discuss SceneCraft: Layout-Guided 3D Scene Generation by Xiuyu Yang, Yunze Man, Jun-Kun Chen, Yu-Xiong Wang. SceneCraft is a method for generating detailed indoor 3D scenes based on user-provided textual descriptions and spatial preferences, using a rendering-based technique and a semantic and depth-conditioned diffusion model to enhance scene representation. It extends beyond single-room creation to design complex multi-room environments like multi-bedroom apartments with diverse layouts. Experimental results demonstrate that SceneCraft outperforms previous techniques in producing intricate and realistic indoor scenes.
Tue, 22 Oct 2024 - 03min - 559 - arxiv preprint - A Tale of Tails: Model Collapse as a Change of Scaling Laws
In this episode, we discuss A Tale of Tails: Model Collapse as a Change of Scaling Laws by Elvis Dohmatob, Yunzhen Feng, Pu Yang, Francois Charton, Julia Kempe. The paper investigates the impact of incorporating synthetic data into training datasets on neural scaling laws and future model performance, questioning whether this integration will lead to continuous improvements or model collapse. It develops a theoretical framework to analyze potential decay phenomena such as loss of scaling and "un-learning" of skills, validated with experiments on arithmetic tasks and text generation. The study underscores the complexity of model success as AI-generated content increases and highlights the need for deeper exploration of models trained on synthesized data from other models.
Fri, 18 Oct 2024 - 04min - 558 - arxiv preprint - Thinking LLMs: General Instruction Following with Thought Generation
In this episode, we discuss Thinking LLMs: General Instruction Following with Thought Generation by Tianhao Wu, Janice Lan, Weizhe Yuan, Jiantao Jiao, Jason Weston, Sainbayar Sukhbaatar. The paper introduces a novel approach to enhance Large Language Models by incorporating an iterative thought process before response generation, which helps in overcoming limitations of current models that lack explicit thinking. This process involves learning through an exploration and optimization framework without needing direct human supervision of thought processes. By employing a judge model for evaluation and preference optimization, the method shows improved performance in reasoning, planning, and other domains such as marketing and health.
Thu, 17 Oct 2024 - 03min - 557 - arxiv preprint - Representation Alignment for Generation: Training Diffusion Transformers Is Easier Than You Think
In this episode, we discuss Representation Alignment for Generation: Training Diffusion Transformers Is Easier Than You Think by Sihyun Yu, Sangkyung Kwak, Huiwon Jang, Jongheon Jeong, Jonathan Huang, Jinwoo Shin, Saining Xie. The paper presents a novel approach called REPresentation Alignment (REPA) to enhance the training efficiency and quality of generative diffusion models by integrating high-quality external visual representations. This method aligns noisy input states with clean image representations from pretrained visual encoders, leading to significantly faster training times—up to 17.5 times faster—and improved generation quality. The results demonstrate that REPA achieves state-of-the-art generation quality using classifier-free guidance compared to traditional methods.
Wed, 16 Oct 2024 - 04min - 556 - arxiv preprint - F5-TTS: A Fairytaler that Fakes Fluent and Faithful Speech with Flow Matching
In this episode, we discuss F5-TTS: A Fairytaler that Fakes Fluent and Faithful Speech with Flow Matching by Yushen Chen, Zhikang Niu, Ziyang Ma, Keqi Deng, Chunhui Wang, Jian Zhao, Kai Yu, Xie Chen. F5-TTS is a fully non-autoregressive text-to-speech system that utilizes flow matching with Diffusion Transformer (DiT) and addresses limitations of previous systems like E2 TTS by padding text inputs with filler tokens to match speech input lengths. It includes ConvNeXt for refining text representations and employs a new Sway Sampling strategy to enhance performance during inference without retraining. The system achieves a rapid inference real-time factor of 0.15 while providing high-quality speech synthesis, capable of zero-shot performance and code-switching, and is trained on a 100K-hour multilingual dataset with resources available for community use.
Mon, 14 Oct 2024 - 04min - 555 - arxiv preprint - One Initialization to Rule them All: Fine-tuning via Explained Variance Adaptation
In this episode, we discuss One Initialization to Rule them All: Fine-tuning via Explained Variance Adaptation by Fabian Paischer, Lukas Hauzenberger, Thomas Schmied, Benedikt Alkin, Marc Peter Deisenroth, Sepp Hochreiter. The paper introduces Explained Variance Adaptation (EVA), a method that enhances the fine-tuning of foundation models by using singular value decomposition for a more effective initialization of LoRA matrices. EVA optimizes rank distribution to capture maximum variance before proceeding with task-specific fine-tuning. This improvement leads to faster convergence and better performance across diverse domains such as language, vision, and reinforcement learning.
Fri, 11 Oct 2024 - 04min - 554 - arxiv preprint - Eliminating Oversaturation and Artifacts of High Guidance Scales in Diffusion Models
In this episode, we discuss Eliminating Oversaturation and Artifacts of High Guidance Scales in Diffusion Models by Seyedmorteza Sadat, Otmar Hilliges, Romann M. Weber. The paper addresses issues with high guidance scales in classifier-free guidance (CFG) for diffusion models, which can cause oversaturation and artifacts. The authors propose a modified update rule by reducing the influence of the parallel component of the update term, leading to a new method called adaptive projected guidance (APG) that maintains quality without oversaturation at higher guidance scales. APG is effective across various models and improves metrics like FID, recall, and saturation, offering a better alternative to standard CFG.
Thu, 10 Oct 2024 - 03min - 553 - arxiv preprint - NEPTUNE: THE LONG ORBIT TO BENCHMARKING LONG VIDEO UNDERSTANDING
In this episode, we discuss NEPTUNE: THE LONG ORBIT TO BENCHMARKING LONG VIDEO UNDERSTANDING by The authors of the paper "NEPTUNE: THE LONG ORBIT TO BENCHMARKING LONG VIDEO UNDERSTANDING" are: - Arsha Nagrani - Mingda Zhang - Ramin Mehran - Rachel Hornung - Nitesh Bharadwaj Gundavarapu - Nilpa Jha - Austin Myers - Xingyi Zhou - Boqing Gong - Cordelia Schmid - Mikhail Sirotenko - Yukun Zhu - Tobias Weyand. The paper introduces "Neptune," a semi-automatic system designed to generate complex question-answer-decoy sets from long video content to enhance comprehension tasks typically limited to short clips. Leveraging large models like Vision-Language Models and Large Language Models, Neptune creates detailed, time-aligned captions and intricate QA sets for videos up to 15 minutes long, aiming to improve annotation efficiency. The dataset emphasizes multimodal reasoning and introduces the GEM metric for evaluating responses, revealing current long video models' weaknesses in understanding temporal and state changes.
Mon, 07 Oct 2024 - 04min - 552 - arxiv preprint - SHIC: Shape-Image Correspondences with no Keypoint Supervision
In this episode, we discuss SHIC: Shape-Image Correspondences with no Keypoint Supervision by Aleksandar Shtedritski, Christian Rupprecht, Andrea Vedaldi. The paper introduces SHIC, a novel method for learning canonical surface mappings without manual supervision by using foundation models such as DINO and Stable Diffusion. SHIC simplifies the task to image-to-image correspondence prediction, outperforming some supervised techniques. The method uses non-photorealistic template renders to effectively simulate manual annotation, allowing reliable canonical map creation for diverse objects.
Fri, 04 Oct 2024 - 03min - 551 - arxiv preprint - E.T. Bench: Towards Open-Ended Event-Level Video-Language Understanding
In this episode, we discuss E.T. Bench: Towards Open-Ended Event-Level Video-Language Understanding by Ye Liu, Zongyang Ma, Zhongang Qi, Yang Wu, Ying Shan, Chang Wen Chen. The paper introduces E.T. Bench, a comprehensive benchmark for fine-grained event-level video understanding, evaluating Video-LLMs across 12 tasks and 7K videos. It highlights the challenges these models face in accurately understanding and grounding events within videos. To improve performance, E.T. Chat and an instruction-tuning dataset, E.T. Instruct 164K, are proposed, enhancing models' abilities and underlining the necessity for advanced datasets and models in temporal and multi-event video-language tasks.
Wed, 02 Oct 2024 - 03min - 550 - arxiv preprint - LLaVA-3D: A Simple yet Effective Pathway to Empowering LMMs with 3D-awareness
In this episode, we discuss LLaVA-3D: A Simple yet Effective Pathway to Empowering LMMs with 3D-awareness by Chenming Zhu, Tai Wang, Wenwei Zhang, Jiangmiao Pang, Xihui Liu. Recent advancements in Large Multimodal Models (LMMs) have significantly improved 2D visual understanding but 3D scene understanding has lagged due to dataset and encoder limitations. The paper introduces LLaVA-3D, a framework that adapts 2D LMMs for 3D understanding by using a 3D Patch representation to link 2D features with 3D positions. This integration allows effective 3D scene understanding without losing 2D capabilities, facilitated by joint 2D and 3D vision-language instruction tuning.
Mon, 30 Sep 2024 - 05min - 549 - arxiv preprint - DepthCrafter: Generating Consistent Long Depth Sequences for Open-world Videos
In this episode, we discuss DepthCrafter: Generating Consistent Long Depth Sequences for Open-world Videos by Wenbo Hu, Xiangjun Gao, Xiaoyu Li, Sijie Zhao, Xiaodong Cun, Yong Zhang, Long Quan, Ying Shan. DepthCrafter is a novel method for estimating temporally consistent depth in open-world videos without needing additional data like camera poses or optical flow. It generalizes to diverse video content by utilizing a three-stage training strategy rooted in a pre-trained image-to-video diffusion model, enabling it to handle up to 110-frame sequences. Evaluations show DepthCrafter's state-of-the-art performance, bolstering applications like depth-based visual effects and conditional video generation.
Fri, 27 Sep 2024 - 04min - 548 - arxiv preprint - Programming Every Example: Lifting Pre-training Data Quality like Experts at Scale
In this episode, we discuss Programming Every Example: Lifting Pre-training Data Quality like Experts at Scale by Fan Zhou, Zengzhi Wang, Qian Liu, Junlong Li, Pengfei Liu. The paper introduces Programming Every Example (PROX), a framework that enables small language models to refine pre-training corpora by executing fine-grained operations on individual examples, outperforming traditional human-crafted rules. Experimental results show that models trained on PROX-curated data achieve over 2% higher performance across various benchmarks compared to other data selection methods. PROX also significantly enhances domain-specific continual pre-training and reduces training FLOPs, with the authors open-sourcing their data and models for further research.
Thu, 26 Sep 2024 - 06min - 547 - arxiv preprint - Phantom of Latent for Large Language and Vision Models
In this episode, we discuss Phantom of Latent for Large Language and Vision Models by Byung-Kwan Lee, Sangyun Chung, Chae Won Kim, Beomchan Park, Yong Man Ro. The paper introduces Phantom, an efficient LLVM family designed to perform comparably to larger models but with significantly smaller sizes, ranging from 0.5B to 7B parameters. By temporarily increasing the latent hidden dimension during multi-head self-attention, Phantom enhances learning capabilities without a substantial increase in model size. Phantom Optimization (PO) combines autoregressive supervised fine-tuning and a direct preference optimization-like concept, resulting in state-of-the-art performance against larger LLVMs.
Tue, 24 Sep 2024 - 05min - 546 - arxiv preprint - Fine-Tuning Image-Conditional Diffusion Models is Easier than You Think
In this episode, we discuss Fine-Tuning Image-Conditional Diffusion Models is Easier than You Think by Gonzalo Martin Garcia, Karim Abou Zeid, Christian Schmidt, Daan de Geus, Alexander Hermans, Bastian Leibe. The study identifies and corrects a flaw in the inference pipeline of large diffusion models used for monocular depth estimation, achieving over 200× speed improvement without compromising accuracy. By end-to-end fine-tuning with task-specific losses, the researchers attain a deterministic model that surpasses all other diffusion-based depth and normal estimation models on zero-shot benchmarks. Moreover, applying this fine-tuning protocol to Stable Diffusion models yields performance comparable to state-of-the-art, challenging prior conclusions in the field.
Fri, 20 Sep 2024 - 05min - 545 - arxiv preprint - On the Diagram of Thought
In this episode, we discuss On the Diagram of Thought by Yifan Zhang, Yang Yuan, Andrew Chi-Chih Yao. Diagram of Thought (DoT) is a framework for modeling iterative reasoning in large language models (LLMs) using a directed acyclic graph (DAG) to organize propositions, critiques, refinements, and verifications. This method allows the model to navigate complex reasoning pathways, improving its logic through natural language feedback via role-specific tokens. DoT also incorporates Topos Theory to ensure logical consistency, enhancing training and inference within a single model without the need for multiple models or external controls.
Thu, 19 Sep 2024 - 03min - 544 - arxiv preprint - Source2Synth: Synthetic Data Generation and Curation Grounded in Real Data Sources
In this episode, we discuss Source2Synth: Synthetic Data Generation and Curation Grounded in Real Data Sources by Alisia Lupidi, Carlos Gemmell, Nicola Cancedda, Jane Dwivedi-Yu, Jason Weston, Jakob Foerster, Roberta Raileanu, Maria Lomeli. The paper presents Source2Synth, a method designed to enhance Large Language Models (LLMs) by generating synthetic data with intermediate reasoning steps, grounded in real-world sources, to improve performance without costly human annotations. Source2Synth also filters out low-quality data points to ensure high-quality datasets. The method demonstrates significant improvements in performance for multi-hop question answering and tool usage in tabular question answering, with respective boosts of 22.57% on HotPotQA and 25.51% on WikiSQL.
Tue, 17 Sep 2024 - 05min - 543 - arxiv preprint - SongCreator: Lyrics-based Universal Song Generation
In this episode, we discuss SongCreator: Lyrics-based Universal Song Generation by Shun Lei, Yixuan Zhou, Boshi Tang, Max W. Y. Lam, Feng Liu, Hangyu Liu, Jingcheng Wu, Shiyin Kang, Zhiyong Wu, Helen Meng. The paper introduces SongCreator, a novel song-generation system designed to create songs with both vocals and accompaniment from given lyrics. This is achieved through a dual-sequence language model (DSLM) and an attention mask strategy, facilitating the model's capability to understand, generate, and edit songs across various tasks. Experiments show that SongCreator achieves state-of-the-art or highly competitive results, particularly excelling in tasks like lyrics-to-song and lyrics-to-vocals, and offers control over acoustic conditions through different prompts.
Thu, 12 Sep 2024 - 04min - 542 - arxiv preprint - Achieving Human Level Competitive Robot Table Tennis
In this episode, we discuss Achieving Human Level Competitive Robot Table Tennis by David B. D'Ambrosio, Saminda Abeyruwan, Laura Graesser, Atil Iscen, Heni Ben Amor, Alex Bewley, Barney J. Reed, Krista Reymann, Leila Takayama, Yuval Tassa, Krzysztof Choromanski, Erwin Coumans, Deepali Jain, Navdeep Jaitly, Natasha Jaques, Satoshi Kataoka, Yuheng Kuang, Nevena Lazic, Reza Mahjourian, Sherry Moore, Kenneth Oslund, Anish Shankar, Vikas Sindhwani, Vincent Vanhoucke, Grace Vesom, Peng Xu, Pannag R. Sanketi. The paper presents a learned robot agent that achieves amateur human-level performance in competitive table tennis by employing a hierarchical and modular policy architecture, including both low-level skill controllers and a high-level decision-making controller. It details techniques for zero-shot sim-to-real transfer and real-time adaptation to new opponents, achieving a 45% win rate in matches against human players of varying skill levels. While the robot consistently won against beginners and intermediates, it lost all matches against advanced players, confirming its amateur performance level.
Wed, 11 Sep 2024 - 03min - 541 - arxiv preprint - Sapiens: Foundation for Human Vision Models
In this episode, we discuss Sapiens: Foundation for Human Vision Models by Rawal Khirodkar, Timur Bagautdinov, Julieta Martinez, Su Zhaoen, Austin James, Peter Selednik, Stuart Anderson, Shunsuke Saito. The Sapiens model family addresses four key human-centric vision tasks and supports 1K high-resolution inference, with easy adaptability through fine-tuning on a large dataset of human images. Self-supervised pretraining significantly enhances performance across these tasks, especially with limited labeled data. Sapiens models achieve state-of-the-art results in benchmarks like Humans-5K, Humans-2K, Hi4D, and THuman2, improving metrics by substantial margins.
Mon, 09 Sep 2024 - 05min - 540 - arxiv preprint - Re-Reading Improves Reasoning in Large Language Models
In this episode, we discuss Re-Reading Improves Reasoning in Large Language Models by Xiaohan Xu, Chongyang Tao, Tao Shen, Can Xu, Hongbo Xu, Guodong Long, Jian-guang Lou. The paper presents a novel prompting method called RE2 (Re-Reading) that improves the reasoning capabilities of Large Language Models by processing questions twice for better understanding. Unlike conventional methods like Chain-of-Thought, RE2 enhances input processing and facilitates bidirectional encoding in unidirectional models. The method demonstrates improved performance across various reasoning benchmarks and shows compatibility and adaptability with different models and prompting strategies.
Fri, 06 Sep 2024 - 04min - 539 - arxiv preprint - SPIRE: Semantic Prompt-Driven Image Restoration
In this episode, we discuss SPIRE: Semantic Prompt-Driven Image Restoration by Chenyang Qi, Zhengzhong Tu, Keren Ye, Mauricio Delbracio, Peyman Milanfar, Qifeng Chen, Hossein Talebi. The paper introduces SPIRE, a novel framework that utilizes semantic and restoration prompts to guide image restoration tasks such as denoising, super-resolution, deblurring, and compression artifact removal. Current text-driven diffusion models excel in general image editing, but SPIRE addresses the gap in fine-level image restoration by incorporating language-based guidance. This approach offers a new paradigm for enhancing image quality through controlled, prompt-driven processes.
Tue, 03 Sep 2024 - 04min - 538 - arxiv preprint - Automated Design of Agentic Systems
In this episode, we discuss Automated Design of Agentic Systems by Shengran Hu, Cong Lu, Jeff Clune. The paper introduces Automated Design of Agentic Systems (ADAS), which aims to replace hand-designed AI solutions with automatically created ones using a new approach where agents are defined and improved by a meta agent through programming. They propose an algorithm called Meta Agent Search, demonstrating its ability to invent novel agent designs that outperform current state-of-the-art models. Their experiments highlight the robustness and generality of these automatically discovered agents across various domains, indicating a promising new direction in AI research.
Fri, 30 Aug 2024 - 04min - 537 - arxiv preprint - Transfusion: Predict the Next Token and Diffuse Images with One Multi-Modal Model
In this episode, we discuss Transfusion: Predict the Next Token and Diffuse Images with One Multi-Modal Model by Chunting Zhou, Lili Yu, Arun Babu, Kushal Tirumala, Michihiro Yasunaga, Leonid Shamis, Jacob Kahn, Xuezhe Ma, Luke Zettlemoyer, Omer Levy. The paper introduces Transfusion, a method for training multi-modal models using a combination of language modeling and diffusion on mixed-modality sequences. Transfusion models, with up to 7B parameters, show superior scaling and performance on uni- and cross-modal benchmarks compared to traditional image token quantization methods. Additionally, the use of modality-specific encoding and decoding layers allows for significant improvements, enabling high-quality image and text generation.
Wed, 28 Aug 2024 - 05min - 536 - arxiv preprint - To Code, or Not To Code? Exploring Impact of Code in Pre-training
In this episode, we discuss To Code, or Not To Code? Exploring Impact of Code in Pre-training by Viraat Aryabumi, Yixuan Su, Raymond Ma, Adrien Morisot, Ivan Zhang, Acyr Locatelli, Marzieh Fadaee, Ahmet Üstün, Sara Hooker. In this study, the impact of incorporating code data during pre-training on various downstream tasks was systematically investigated. The findings indicate that including code enhances performance in natural language reasoning, world knowledge, and code-specific tasks, suggesting that code data is essential for generalization beyond just coding tasks. Specifically, code inclusion resulted in significant performance improvements, highlighting the importance of maintaining high-quality code data in pre-training LLMs.
Mon, 26 Aug 2024 - 04min - 535 - arxiv preprint - Segment Anything with Multiple Modalities
In this episode, we discuss Segment Anything with Multiple Modalities by Aoran Xiao, Weihao Xuan, Heli Qi, Yun Xing, Naoto Yokoya, Shijian Lu. The paper introduces MM-SAM, an extension of the Segment Anything Model (SAM) tailored for multi-modal data from various sensor suites, such as LiDAR plus RGB and thermal plus RGB. MM-SAM employs unsupervised cross-modal transfer and weakly-supervised multi-modal fusion to adapt efficiently to different sensor modalities. Extensive experiments validate that MM-SAM significantly outperforms the original SAM in robustness and segmentation accuracy across various sensors and modalities.
Fri, 23 Aug 2024 - 05min - 534 - arxiv preprint - JPEG-LM: LLMs as Image Generators with Canonical Codec Representations
In this episode, we discuss JPEG-LM: LLMs as Image Generators with Canonical Codec Representations by Xiaochuang Han, Marjan Ghazvininejad, Pang Wei Koh, Yulia Tsvetkov. The paper introduces a novel approach for image and video generation by modeling them as compressed files using standard codecs like JPEG and AVC/H.264. Instead of pixel-based or vector quantization methods, the authors employ the Llama architecture to directly output the compressed bytes, showing improved performance and simplicity. This method achieves a significant reduction in FID and excels in generating long-tail visual elements, highlighting its potential for seamless integration into multimodal systems.
Tue, 20 Aug 2024 - 04min - 533 - arxiv preprint - Mission: Impossible Language Models
In this episode, we discuss Mission: Impossible Language Models by Julie Kallini, Isabel Papadimitriou, Richard Futrell, Kyle Mahowald, Christopher Potts. The paper investigates Chomsky's claim that large language models (LLMs) can learn both possible and impossible languages by designing synthetic impossible languages with unnatural word orders and grammar rules. Experiments conducted using GPT-2 small models reveal that these models struggle to learn such impossible languages compared to English, challenging the initial claim. The study aims to inspire further research into testing various LLM architectures on impossible languages to better understand their cognitive and typological implications.
Mon, 19 Aug 2024 - 05min - 532 - arxiv preprint - Learning Task Decomposition to Assist Humans in Competitive Programming
In this episode, we discuss Learning Task Decomposition to Assist Humans in Competitive Programming by Jiaxin Wen, Ruiqi Zhong, Pei Ke, Zhihong Shao, Hongning Wang, Minlie Huang. The paper presents a method to enhance human understanding and repair of language model (LM)-generated solutions by automatically breaking down complex solutions into simpler subtasks. They introduce a novel objective called assistive value (AssistV) to measure how easily humans can repair these subtasks and validate their method through a dataset of human repair experiences. The approach significantly improves the problem-solving ability and speed of non-experts in competitive programming, allowing them to solve more problems and match the performance of unassisted experts.
Fri, 16 Aug 2024 - 05min - 531 - arxiv preprint - IPAdapter-Instruct: Resolving Ambiguity in Image-based Conditioning using Instruct Prompts
In this episode, we discuss IPAdapter-Instruct: Resolving Ambiguity in Image-based Conditioning using Instruct Prompts by Ciara Rowles, Shimon Vainer, Dante De Nigris, Slava Elizarov, Konstantin Kutsy, Simon Donné. The paper discusses IPAdapter-Instruct, a method combining natural-image conditioning with "Instruct" prompts to enable nuanced control over image generation. This approach allows for multiple interpretations (like style transfer or object extraction) of the same conditioning image, addressing limitations of current models that require multiple adapters for different tasks. IPAdapter-Instruct effectively learns various tasks with minimal quality loss, enhancing practical usability in workflows requiring diverse outputs.
Tue, 13 Aug 2024 - 04min - 530 - arxiv preprint - Scaling LLM Test-Time Compute Optimally can be More Effective than Scaling Model Parameters
In this episode, we discuss Scaling LLM Test-Time Compute Optimally can be More Effective than Scaling Model Parameters by Charlie Snell, Jaehoon Lee, Kelvin Xu, Aviral Kumar. The paper explores the impact of increased inference-time computation on Large Language Models (LLMs) to enhance their performance on challenging prompts. It examines two primary methods for scaling test-time computation and finds that their effectiveness varies with the prompt's difficulty, advocating for an adaptive “compute-optimal” strategy. This approach significantly improves test-time compute efficiency and can enable smaller models to outperform much larger ones under computationally equivalent conditions.
Sat, 10 Aug 2024 - 04min - 529 - arxiv preprint - Language Model Can Listen While Speaking
In this episode, we discuss Language Model Can Listen While Speaking by Ziyang Ma, Yakun Song, Chenpeng Du, Jian Cong, Zhuo Chen, Yuping Wang, Yuxuan Wang, Xie Chen. The paper explores enhancing real-time interaction in speech-based conversational AI by introducing listening-while-speaking language models (LSLM) for full duplex communication. LSLM integrates simultaneous listening and speaking capabilities using a token-based decoder-only TTS and a streaming SSL encoder. Experimental results show LSLM's robustness and sensitivity to diverse instructions, advocating its potential to improve interactive speech dialogue systems in real-world applications.
Thu, 08 Aug 2024 - 04min - 528 - arxiv preprint - Improving Text Embeddings for Smaller Language Models Using Contrastive Fine-tuning
In this episode, we discuss Improving Text Embeddings for Smaller Language Models Using Contrastive Fine-tuning by Trapoom Ukarapol, Zhicheng Lee, Amy Xin. The paper investigates enhancing smaller language models, like MiniCPM, through improved text embeddings via contrastive fine-tuning on the NLI dataset. Results indicate that this fine-tuning significantly improves performance across multiple benchmarks, with MiniCPM showing a notable 56.33% performance gain. The study's code is available at https://github.com/trapoom555/Language-Model-STS-CFT.
Wed, 07 Aug 2024 - 05min - 527 - arxiv preprint - Cycle3D: High-quality and Consistent Image-to-3D Generation via Generation-Reconstruction Cycle
In this episode, we discuss Cycle3D: High-quality and Consistent Image-to-3D Generation via Generation-Reconstruction Cycle by Zhenyu Tang, Junwu Zhang, Xinhua Cheng, Wangbo Yu, Chaoran Feng, Yatian Pang, Bin Lin, Li Yuan. Recent 3D large reconstruction models often generate low-quality and inconsistent multi-view images, which harm the final 3D output. To resolve this, the proposed Cycle3D framework integrates a 2D diffusion-based generation module and a 3D reconstruction module to iteratively enhance texture quality and multi-view consistency. Experiments show that Cycle3D outperforms state-of-the-art methods in creating high-quality and consistent 3D content.
Tue, 06 Aug 2024 - 04min - 526 - arxiv preprint - Towards Achieving Human Parity on End-to-end Simultaneous Speech Translation via LLM Agent
In this episode, we discuss Towards Achieving Human Parity on End-to-end Simultaneous Speech Translation via LLM Agent by Shanbo Cheng, Zhichao Huang, Tom Ko, Hang Li, Ningxin Peng, Lu Xu, Qini Zhang. The paper introduces CLASI, a high-quality and human-like Simultaneous Speech Translation (SiST) system inspired by professional interpreters' strategies to balance translation quality and latency. Utilizing a multi-modal retrieving module and Large Language Models (LLMs), CLASI significantly outperforms other systems, especially in challenging real-world scenarios. Evaluated using the valid information proportion (VIP) metric, CLASI achieves impressive results compared to state-of-the-art systems, with VIP scores of 81.3% for Chinese-to-English and 78.0% for English-to-Chinese translations.
Tue, 06 Aug 2024 - 04min - 525 - arxiv preprint - Graph-enhanced Large Language Models in Asynchronous Plan Reasoning
In this episode, we discuss Graph-enhanced Large Language Models in Asynchronous Plan Reasoning by Fangru Lin, Emanuele La Malfa, Valentin Hofmann, Elle Michelle Yang, Anthony Cohn, Janet B. Pierrehumbert. The paper investigates how well large language models (LLMs) like GPT-4 and LLaMA-2 handle reasoning about asynchronous plans and finds that they perform poorly without visual aids. It introduces a new technique, Plan Like a Graph (PLaG), which integrates graphs with language prompts, significantly improving model performance. Despite this improvement, the study highlights the limitations of LLMs when dealing with complex tasks, underscoring the challenges of using them as autonomous agents.
Wed, 31 Jul 2024 - 03min - 524 - arxiv preprint - LazyLLM: Dynamic Token Pruning for Efficient Long Context LLM Inference
In this episode, we discuss LazyLLM: Dynamic Token Pruning for Efficient Long Context LLM Inference by Qichen Fu, Minsik Cho, Thomas Merth, Sachin Mehta, Mohammad Rastegari, Mahyar Najibi. The paper introduces LazyLLM, a method that selectively computes only the essential token's Key-Value (KV) cache for next token prediction during the prefilling and decoding stages of transformer-based language models to address the bottleneck caused by long prompts. Unlike static pruning approaches, LazyLLM dynamically adapts which tokens to consider at each generation step. This method significantly accelerates the generation process without sacrificing accuracy, as demonstrated in experiments like the multi-document question-answering task with LLama 2 7B model, achieving a 2.34× speedup.
Tue, 30 Jul 2024 - 03min - 523 - arxiv preprint - OutfitAnyone: Ultra-high Quality Virtual Try-On for Any Clothing and Any Person
In this episode, we discuss OutfitAnyone: Ultra-high Quality Virtual Try-On for Any Clothing and Any Person by Ke Sun, Jian Cao, Qi Wang, Linrui Tian, Xindi Zhang, Lian Zhuo, Bang Zhang, Liefeng Bo, Wenbo Zhou, Weiming Zhang, Daiheng Gao. Virtual Try-On (VTON) technology faces challenges in generating high-fidelity and consistent images. While existing diffusion models struggle with control in VTON scenarios, OutfitAnyone uses a two-stream conditional diffusion model to overcome these issues, achieving lifelike results and scalability across various scenarios. This method effectively handles garment deformation and adapts to different poses, body shapes, and image types, making it suitable for real-world applications.
Mon, 29 Jul 2024 - 04min - 522 - arxiv preprint - DetToolChain: A New Prompting Paradigm to Unleash Detection Ability of MLLM
In this episode, we discuss DetToolChain: A New Prompting Paradigm to Unleash Detection Ability of MLLM by Yixuan Wu, Yizhou Wang, Shixiang Tang, Wenhao Wu, Tong He, Wanli Ouyang, Philip Torr, Jian Wu. DetToolChain introduces a prompting toolkit and a Chain-of-Thought methodology to enhance zero-shot object detection capabilities in multimodal large language models like GPT-4V and Gemini. The toolkit employs precise detection strategies and tools such as zooming, overlaying rulers, and scene graphs to help the models focus and infer better. Experimental results demonstrate significant performance improvements in various detection tasks, surpassing state-of-the-art methods considerably.
Fri, 26 Jul 2024 - 04min - 521 - arxiv preprint - Conditioned Language Policy: A General Framework for Steerable Multi-Objective Finetuning
In this episode, we discuss Conditioned Language Policy: A General Framework for Steerable Multi-Objective Finetuning by Kaiwen Wang, Rahul Kidambi, Ryan Sullivan, Alekh Agarwal, Christoph Dann, Andrea Michi, Marco Gelmi, Yunxuan Li, Raghav Gupta, Avinava Dubey, Alexandre Ramé, Johan Ferret, Geoffrey Cideron, Le Hou, Hongkun Yu, Amr Ahmed, Aranyak Mehta, Léonard Hussenot, Olivier Bachem, Edouard Leurent. The paper presents Conditioned Language Policies (CLP), a framework for finetuning language models to balance multiple conflicting objectives. CLP leverages multi-task training and parameter-efficient finetuning to allow a single model to navigate trade-offs between objectives during inference. Experiments show that CLP outperforms existing methods, making it a superior approach for creating steerable and flexible language models.
Tue, 23 Jul 2024 - 04min - 520 - arxiv preprint - Chameleon: Mixed-Modal Early-Fusion Foundation Models
In this episode, we discuss Chameleon: Mixed-Modal Early-Fusion Foundation Models by Chameleon Team. The paper introduces Chameleon, a family of models designed for seamless understanding and generating both images and text in any sequence. It achieves state-of-the-art performance in several tasks, including image captioning and text generation, and demonstrates competence in mixed-modal outputs. Notably, Chameleon is competitive with or superior to larger models like Gemini Pro and GPT-4V in various evaluations, highlighting its significance in multimodal document processing.
Mon, 22 Jul 2024 - 04min - 519 - arxiv preprint - Goldfish: Vision-Language Understanding of Arbitrarily Long Videos
In this episode, we discuss Goldfish: Vision-Language Understanding of Arbitrarily Long Videos by Kirolos Ataallah, Xiaoqian Shen, Eslam Abdelrahman, Essam Sleiman, Mingchen Zhuge, Jian Ding, Deyao Zhu, Jürgen Schmidhuber, Mohamed Elhoseiny. The paper introduces Goldfish, a methodology designed to efficiently comprehend videos of any length by employing a retrieval mechanism that selects top-k relevant video clips for processing. To evaluate its effectiveness, the authors present the TVQA-long benchmark aimed at long video understanding and demonstrate significant improvements over existing methods, achieving a 41.78% accuracy rate. Additionally, their MiniGPT4-Video model also excels in short video comprehension, outperforming current state-of-the-art methods on multiple benchmarks.
Thu, 18 Jul 2024 - 04min - 518 - arxiv preprint - Masked Generative Video-to-Audio Transformers with Enhanced Synchronicity
In this episode, we discuss Masked Generative Video-to-Audio Transformers with Enhanced Synchronicity by Santiago Pascual, Chunghsin Yeh, Ioannis Tsiamas, Joan Serrà. The paper introduces MaskVAT, a video-to-audio generative model that utilizes a masked generative model alongside a high-quality general audio codec to achieve superior audio quality, semantic matching, and temporal synchronization. MaskVAT effectively addresses the synchronization issues in previous V2A models without compromising on audio quality. Empirical results demonstrate its capability to generate well-synchronized and high-quality audio that aligns with visual actions, competing with state-of-the-art non-codec generative models.
Wed, 17 Jul 2024 - 03min - 517 - arxiv preprint - Human-like Episodic Memory for Infinite Context LLMs
In this episode, we discuss Human-like Episodic Memory for Infinite Context LLMs by Zafeirios Fountas, Martin A Benfeghoul, Adnan Oomerjee, Fenia Christopoulou, Gerasimos Lampouras, Haitham Bou-Ammar, Jun Wang. The paper introduces EM-LLM, an approach that enhances large language models (LLMs) by incorporating principles of human episodic memory and event cognition, enabling them to manage extensive contexts efficiently. EM-LLM uses Bayesian surprise and graph-theoretic boundary refinement to organize token sequences into episodic events and employs a two-stage memory process for effective retrieval. Experiments demonstrate that EM-LLM outperforms existing models on various tasks, showing significant improvement, and aligning well with human event perception, suggesting potential for interdisciplinary AI and cognitive science research.
Mon, 15 Jul 2024 - 05min - 516 - arxiv preprint - Learning to (Learn at Test Time): RNNs with Expressive Hidden States
In this episode, we discuss Learning to (Learn at Test Time): RNNs with Expressive Hidden States by Yu Sun, Xinhao Li, Karan Dalal, Jiarui Xu, Arjun Vikram, Genghan Zhang, Yann Dubois, Xinlei Chen, Xiaolong Wang, Sanmi Koyejo, Tatsunori Hashimoto, Carlos Guestrin. The paper introduces Test-Time Training (TTT) layers, a new type of sequence modeling layer combining the efficiency of RNNs with the long-context performance of self-attention mechanisms. TTT layers make use of a machine learning model as their hidden state, updated through self-supervised learning iterations even on test sequences. The proposed TTT-Linear and TTT-MLP models demonstrate competitive or superior performance to both advanced Transformers and modern RNNs like Mamba, with TTT-Linear proving more efficient in certain long-context scenarios.
Fri, 12 Jul 2024 - 04min - 515 - arxiv preprint - Graph-Based Captioning: Enhancing Visual Descriptions by Interconnecting Region Captions
In this episode, we discuss Graph-Based Captioning: Enhancing Visual Descriptions by Interconnecting Region Captions by Yu-Guan Hsieh, Cheng-Yu Hsieh, Shih-Ying Yeh, Louis Béthune, Hadi Pour Ansari, Pavan Kumar Anasosalu Vasu, Chun-Liang Li, Ranjay Krishna, Oncel Tuzel, Marco Cuturi. The paper introduces a new annotation strategy termed graph-based captioning (GBC) that uses labelled graph structures to describe images more richly than plain text. GBC combines object detection and dense captioning to create a hierarchical graph of nodes and edges detailing entities and their relationships. The authors demonstrate the effectiveness of GBC by creating a large dataset, GBC10M, which significantly improves performance in vision-language models and propose a novel attention mechanism to utilize the graph's structure for further benefits.
Thu, 11 Jul 2024 - 04min - 514 - arxiv preprint - Evaluating Human Alignment and Model Faithfulness of LLM Rationale
In this episode, we discuss Evaluating Human Alignment and Model Faithfulness of LLM Rationale by Mohsen Fayyaz, Fan Yin, Jiao Sun, Nanyun Peng. The paper investigates how effectively large language models (LLMs) can explain their decisions through rationales extracted from input texts. It compares two types of rationale extraction methods—attribution-based and prompting-based—finding that prompting-based rationales better align with human-annotated rationales. The study also explores the faithfulness limitations of prompting-based methods and shows that fine-tuning models on specific datasets can improve the faithfulness of both rationale extraction approaches.
Tue, 09 Jul 2024 - 04min - 513 - arxiv preprint - Detection and Measurement of Syntactic Templates in Generated Text
In this episode, we discuss Detection and Measurement of Syntactic Templates in Generated Text by Chantal Shaib, Yanai Elazar, Junyi Jessy Li, Byron C. Wallace. The paper investigates syntactic features in text generated by large language models (LLMs), revealing higher rates of templated text in these models compared to human-generated text. It finds that a significant portion of these templates originates from pre-training data and remain unchanged during fine-tuning. The study demonstrates that syntactic templates can distinguish between different models and tasks, and serves as an effective tool for evaluating style memorization in LLMs.
Mon, 08 Jul 2024 - 05min - 512 - arxiv preprint - From Artificial Needles to Real Haystacks: Improving Retrieval Capabilities in LLMs by Finetuning on Synthetic Data
In this episode, we discuss From Artificial Needles to Real Haystacks: Improving Retrieval Capabilities in LLMs by Finetuning on Synthetic Data by Zheyang Xiong, Vasilis Papageorgiou, Kangwook Lee, Dimitris Papailiopoulos. This paper addresses the challenge Large Language Models (LLMs) face with long-context information retrieval and reasoning. The authors propose finetuning LLMs using a synthetic dataset designed for numerical key-value retrieval tasks, resulting in significant improvements. Experiments demonstrate enhanced performance on longer-context tasks without compromising general benchmark performance, unlike other long-context augmentation methods that can provoke hallucination.
Mon, 01 Jul 2024 - 05min - 511 - arxiv preprint - MG-LLaVA: Towards Multi-Granularity Visual Instruction Tuning
In this episode, we discuss MG-LLaVA: Towards Multi-Granularity Visual Instruction Tuning by Xiangyu Zhao, Xiangtai Li, Haodong Duan, Haian Huang, Yining Li, Kai Chen, Hua Yang. The study presents MG-LLaVA, a multi-modal large language model designed to process both low-resolution and high-resolution images along with object-centric features for improved perception tasks. It includes a high-resolution visual encoder and a Conv-Gate fusion network to amalgamate fine-grained details with base features, enhancing object recognition using bounding box-derived data from offline detectors. Extensive benchmarking demonstrates MG-LLaVA's superior performance over comparable MLLMs, validated by evaluations using various language encoders ranging from 3.8B to 34B parameters.
Thu, 27 Jun 2024 - 06min - 510 - arxiv preprint - 4M-21: An Any-to-Any Vision Model for Tens of Tasks and Modalities
In this episode, we discuss 4M-21: An Any-to-Any Vision Model for Tens of Tasks and Modalities by Roman Bachmann, Oğuzhan Fatih Kar, David Mizrahi, Ali Garjani, Mingfei Gao, David Griffiths, Jiaming Hu, Afshin Dehghan, Amir Zamir. The paper presents a novel any-to-any model that significantly extends the capabilities of existing multimodal and multitask foundation models by training on tens of highly diverse modalities, including images, text, geometric data, and more. Through discrete tokenization of various data types and co-training on large-scale datasets, the model can address three times more tasks/modalities than current models without sacrificing performance. The authors demonstrate this with a three billion parameter model, providing open access to the models and training code.
Wed, 26 Jun 2024 - 04min - 509 - arxiv preprint - VideoLLM-online: Online Video Large Language Model for Streaming Video
In this episode, we discuss VideoLLM-online: Online Video Large Language Model for Streaming Video by Joya Chen, Zhaoyang Lv, Shiwei Wu, Kevin Qinghong Lin, Chenan Song, Difei Gao, Jia-Wei Liu, Ziteng Gao, Dongxing Mao, Mike Zheng Shou. The paper discusses the development of the Learning-In-Video-Stream (LIVE) framework, which improves large multimodal models' ability to handle real-time streaming video inputs. The framework includes a training objective for continuous input, data generation for streaming dialogue, and an optimized inference pipeline, leading to enhanced performance and speed. This innovation, demonstrated through the VideoLLM-online model built on Llama-2/Llama-3, shows significant improvements in handling streaming videos and achieves state-of-the-art performance in various video-related tasks.
Tue, 25 Jun 2024 - 04min - 508 - arxiv preprint - EvTexture: Event-driven Texture Enhancement for Video Super-Resolution
In this episode, we discuss EvTexture: Event-driven Texture Enhancement for Video Super-Resolution by Dachun Kai, Jiayao Lu, Yueyi Zhang, Xiaoyan Sun. The paper introduces EvTexture, the first video super-resolution (VSR) method using event signals specifically for enhancing texture details. The proposed method employs a new texture enhancement branch and an iterative module to progressively refine textures, leveraging the high-frequency details from event data. Experimental results demonstrate that EvTexture achieves state-of-the-art performance, significantly improving resolution and detail on datasets especially rich in textures.
Mon, 24 Jun 2024 - 05min - 507 - arxiv preprint - MOFA-Video: Controllable Image Animation via Generative Motion Field Adaptions in Frozen Image-to-Video Diffusion Model
In this episode, we discuss MOFA-Video: Controllable Image Animation via Generative Motion Field Adaptions in Frozen Image-to-Video Diffusion Model by Muyao Niu, Xiaodong Cun, Xintao Wang, Yong Zhang, Ying Shan, Yinqiang Zheng. MOFA-Video is a novel image animation technique that produces videos from a single image using various control signals like human landmarks, manual trajectories, or another video. Unlike previous methods limited to specific motion domains or with weak control capabilities, MOFA-Video employs domain-aware motion field adapters (MOFA-Adapters) to manage generated motions. These adapters ensure temporal motion consistency by converting sparse control inputs into dense motion flows at multiple scales.
Fri, 21 Jun 2024 - 05min - 506 - arxiv preprint - An Image is Worth More Than 16x16 Patches: Exploring Transformers on Individual Pixels
In this episode, we discuss An Image is Worth More Than 16x16 Patches: Exploring Transformers on Individual Pixels by Duy-Kien Nguyen, Mahmoud Assran, Unnat Jain, Martin R. Oswald, Cees G. M. Snoek, Xinlei Chen. This paper questions the necessity of locality inductive bias in modern computer vision architectures by showing that vanilla Transformers can treat each individual pixel as a token and still achieve high performance. The authors demonstrate this across three tasks: object classification, self-supervised learning via masked autoencoding, and image generation with diffusion models. Despite its computational inefficiency, this finding suggests reconsidering design principles for future neural architectures in computer vision.
Thu, 20 Jun 2024 - 05min - 505 - arxiv preprint - Graphic Design with Large Multimodal Model
In this episode, we discuss Graphic Design with Large Multimodal Model by Yutao Cheng, Zhao Zhang, Maoke Yang, Hui Nie, Chunyuan Li, Xinglong Wu, Jie Shao. The paper introduces Hierarchical Layout Generation (HLG) for graphic design, which creates compositions from unordered sets of design elements, addressing limitations of the existing Graphic Layout Generation (GLG). The authors develop Graphist, a novel layout generation model that uses large multimodal models to translate RGB-A images into a JSON draft protocol specifying the design layout's details. Graphist demonstrates superior performance compared to prior models and establishes a new baseline for HLG, complemented by the introduction of multiple evaluation metrics.
Wed, 19 Jun 2024 - 03min - 504 - arxiv preprint - LLARVA: Vision-Action Instruction Tuning Enhances Robot Learning
In this episode, we discuss LLARVA: Vision-Action Instruction Tuning Enhances Robot Learning by Dantong Niu, Yuvan Sharma, Giscard Biamby, Jerome Quenum, Yutong Bai, Baifeng Shi, Trevor Darrell, Roei Herzig. The paper introduces LLARVA, a model improved with a novel instruction-tuning method to unify various robotic tasks using structured prompts. The model utilizes 2-D visual traces to better align vision and action spaces, pre-trained on 8.5M image-visual trace pairs from the Open X-Embodiment dataset. Experiments on the RLBench simulator and a physical robot demonstrate that LLARVA outperforms several baselines and generalizes well across different robotic environments.
Tue, 18 Jun 2024 - 04min - 503 - arxiv preprint - Transformers need glasses! Information over-squashing in language tasks
In this episode, we discuss Transformers need glasses! Information over-squashing in language tasks by Federico Barbero, Andrea Banino, Steven Kapturowski, Dharshan Kumaran, João G. M. Araújo, Alex Vitvitskyi, Razvan Pascanu, Petar Veličković. The paper explores how information propagates in decoder-only Transformers, revealing a phenomenon where different input sequences can result in nearly identical final token representations. This issue, worsened by low-precision floating-point formats, impairs the model’s ability to distinguish between these sequences, leading to errors in specific tasks. The authors provide theoretical and empirical evidence of this problem and suggest simple solutions to mitigate it.
Mon, 17 Jun 2024 - 04min - 502 - arxiv preprint - Show, Don’t Tell: Aligning Language Models with Demonstrated Feedback
In this episode, we discuss Show, Don't Tell: Aligning Language Models with Demonstrated Feedback by Omar Shaikh, Michelle Lam, Joey Hejna, Yijia Shao, Michael Bernstein, Diyi Yang. The paper introduces Demonstration ITerated Task Optimization (DITTO), a method for customizing language model outputs using fewer than ten demonstrations as feedback. DITTO, based on online imitation learning, aligns the model's outputs to user-specific behavior by generating comparison data iteratively. DITTO outperforms existing methods like few-shot prompting and supervised fine-tuning by an average of 19% in matching fine-grained styles and tasks.
Fri, 14 Jun 2024 - 05min - 501 - arxiv preprint - TextGrad: Automatic ”Differentiation” via Text
In this episode, we discuss TextGrad: Automatic "Differentiation" via Text by Mert Yuksekgonul, Federico Bianchi, Joseph Boen, Sheng Liu, Zhi Huang, Carlos Guestrin, James Zou. The paper introduces TEXTGRAD, a novel framework that automates the optimization of compound AI systems by utilizing textual feedback from large language models (LLMs). TEXTGRAD treats text feedback as a form of "differentiation" to improve the components of these AI systems across various applications, working out-of-the-box without requiring specific tuning. Demonstrating its effectiveness, TEXTGRAD enhances performance in diverse tasks such as question answering, coding problem solutions, molecule design, and treatment planning, marking a significant step forward for the development of advanced AI technologies.
Thu, 13 Jun 2024 - 04min - 500 - arxiv preprint - SaySelf: Teaching LLMs to Express Confidence with Self-Reflective Rationales
In this episode, we discuss SaySelf: Teaching LLMs to Express Confidence with Self-Reflective Rationales by Tianyang Xu, Shujin Wu, Shizhe Diao, Xiaoze Liu, Xingyao Wang, Yangyi Chen, Jing Gao. The paper introduces SaySelf, a framework for training large language models (LLMs) to produce accurate, fine-grained confidence estimates and self-reflective rationales explaining their uncertainties. This is achieved by analyzing inconsistencies in multiple reasoning chains, summarizing uncertainties in natural language, and applying supervised fine-tuning alongside reinforcement learning to calibrate confidence levels. Experimental results show that SaySelf effectively reduces confidence calibration errors and maintains task performance, enhancing LLMs' reliability by mitigating overconfidence in erroneous outputs.
Wed, 12 Jun 2024 - 04min - 499 - arxiv preprint - Open-Endedness is Essential for Artificial Superhuman Intelligence
In this episode, we discuss Open-Endedness is Essential for Artificial Superhuman Intelligence by Edward Hughes, Michael Dennis, Jack Parker-Holder, Feryal Behbahani, Aditi Mavalankar, Yuge Shi, Tom Schaul, Tim Rocktaschel. The paper argues that the development of open-ended, self-improving AI systems is achievable using current foundation models trained on extensive internet data. It provides a formal definition of open-endedness based on novelty and learnability and suggests a path to artificial superhuman intelligence (ASI) through such systems. The paper emphasizes the importance of considering safety in the development of these highly capable and open-ended AI systems.
Tue, 11 Jun 2024 - 04min - 498 - arxiv preprint - To Believe or Not to Believe Your LLM
In this episode, we discuss To Believe or Not to Believe Your LLM by Yasin Abbasi Yadkori, Ilja Kuzborskij, András György, Csaba Szepesvári. The study investigates uncertainty quantification in large language models (LLMs), focusing on distinguishing large epistemic uncertainty to identify unreliable outputs and potential hallucinations. By employing an information-theoretic metric and a method of iterative prompting based on prior responses, the approach effectively detects high uncertainty scenarios, particularly in distinguishing between cases with single and multiple possible answers. The proposed method outperforms standard strategies and highlights how iterative prompting influences the probability assignments of LLM outputs.
Fri, 07 Jun 2024 - 04min - 497 - arxiv preprint - Similarity is Not All You Need: Endowing Retrieval Augmented Generation with Multi Layered Thoughts
In this episode, we discuss Similarity is Not All You Need: Endowing Retrieval Augmented Generation with Multi Layered Thoughts by Chunjing Gan, Dan Yang, Binbin Hu, Hanxiao Zhang, Siyuan Li, Ziqi Liu, Yue Shen, Lin Ju, Zhiqiang Zhang, Jinjie Gu, Lei Liang, Jun Zhou. The paper introduces METRAG, a novel Multi-layered Thought enhanced Retrieval-Augmented Generation framework designed to improve the performance of LLMs in knowledge-intensive tasks. Unlike traditional models that solely rely on similarity for document retrieval, METRAG combines similarity-oriented, utility-oriented, and compactness-oriented thoughts to enhance the retrieval and generation process. The framework has shown superior results in various experiments, addressing concerns about knowledge update delays, cost, and hallucinations in LLMs.
Wed, 05 Jun 2024 - 04min - 496 - arxiv preprint - Contextual Position Encoding: Learning to Count What’s Important
In this episode, we discuss Contextual Position Encoding: Learning to Count What's Important by Olga Golovneva, Tianlu Wang, Jason Weston, Sainbayar Sukhbaatar. The paper introduces Contextual Position Encoding (CoPE), a new position encoding method for Large Language Models (LLMs) that incrementally alters position based on context rather than just token count. This approach enables more sophisticated addressing, such as targeting specific types of words or sentences, beyond the capabilities of current token-based methods. Through experiments, CoPE demonstrates improved performance on tasks like selective copy, counting, and Flip-Flop, as well as enhancements in language modeling and coding task perplexity.
Tue, 04 Jun 2024 - 04min - 495 - arxiv preprint - Video-MME: The First-Ever Comprehensive Evaluation Benchmark of Multi-modal LLMs in Video Analysis
In this episode, we discuss Video-MME: The First-Ever Comprehensive Evaluation Benchmark of Multi-modal LLMs in Video Analysis by Chaoyou Fu, Yuhan Dai, Yondong Luo, Lei Li, Shuhuai Ren, Renrui Zhang, Zihan Wang, Chenyu Zhou, Yunhang Shen, Mengdan Zhang, Peixian Chen, Yanwei Li, Shaohui Lin, Sirui Zhao, Ke Li, Tong Xu, Xiawu Zheng, Enhong Chen, Rongrong Ji, Xing Sun. The paper introduces Video-MME, a comprehensive benchmark for evaluating Multi-modal Large Language Models (MLLMs) in video analysis, which assesses capabilities across diverse video types, durations, and data modalities with high-quality annotations. Their experiments show commercial models like Gemini 1.5 Pro outperform open-source counterparts and highlight the significant impact of subtitles and audio on video understanding, along with a noted drop in model performance with longer videos. The findings emphasize the need for improvements in handling extended sequences and multi-modal data, driving future advancements in MLLM capabilities.
Mon, 03 Jun 2024 - 05min - 494 - arxiv preprint - VideoTree: Adaptive Tree-based Video Representation for LLM Reasoning on Long Videos
In this episode, we discuss VideoTree: Adaptive Tree-based Video Representation for LLM Reasoning on Long Videos by Ziyang Wang, Shoubin Yu, Elias Stengel-Eskin, Jaehong Yoon, Feng Cheng, Gedas Bertasius, Mohit Bansal. The paper introduces VideoTree, a novel framework that enhances the efficiency and accuracy of long-video question answering by selectively extracting and hierarchically organizing frames based on their relevance to the query. Unlike traditional methods that rely on dense and often redundant sampling of frames for LLM-based reasoning, VideoTree employs a dynamic, adaptive approach to identify and caption keyframes, forming a tree structure that reflects varying levels of detail where needed. Experiments demonstrate significant performance improvements and reduced inference times on benchmarks like EgoSchema, NExT-QA, and IntentQA.
Fri, 31 May 2024 - 04min - 493 - arxiv preprint - CinePile: A Long Video Question Answering Dataset and Benchmark
In this episode, we discuss CinePile: A Long Video Question Answering Dataset and Benchmark by Ruchit Rawal, Khalid Saifullah, Ronen Basri, David Jacobs, Gowthami Somepalli, Tom Goldstein. CinePile is a new dataset and benchmark designed for authentic long-form video understanding, addressing the limitations of current datasets. It comprises 305,000 multiple-choice questions (MCQs) spanning various visual and multimodal aspects. The evaluation of recent state-of-the-art video-centric language models (LLMs) shows a significant gap between machine and human performance in these complex tasks.
Thu, 30 May 2024 - 05min - 492 - arxiv preprint - Dataset Decomposition: Faster LLM Training with Variable Sequence Length Curriculum
In this episode, we discuss Dataset Decomposition: Faster LLM Training with Variable Sequence Length Curriculum by Hadi Pouransari, Chun-Liang Li, Jen-Hao Rick Chang, Pavan Kumar Anasosalu Vasu, Cem Koc, Vaishaal Shankar, Oncel Tuzel. The paper introduces a novel variable sequence length training technique called dataset decomposition to address inefficiencies in training large language models (LLMs) with fixed-length token sequences. It divides the dataset into buckets of sequences of the same size from unique documents and samples from these buckets with a curriculum during training, leading to computational savings and higher efficiency. This approach achieves target accuracy three times faster than traditional methods and enhances performance on standard language evaluations and long-context benchmarks.
Wed, 29 May 2024 - 05min - 491 - arxiv preprint - SWE-agent: Agent-Computer Interfaces Enable Automated Software Engineering
In this episode, we discuss SWE-agent: Agent-Computer Interfaces Enable Automated Software Engineering by John Yang, Carlos E. Jimenez, Alexander Wettig, Kilian Lieret, Shunyu Yao, Karthik Narasimhan, Ofir Press. The paper introduces SWE-agent, an autonomous system leveraging a language model to tackle software engineering tasks through a specialized agent-computer interface (ACI). SWE-agent significantly improves task completion rates, solving 12.5% of issues on SWE-bench compared to the previous best of 3.8%. The study also examines the impact of ACI design on agent performance, offering insights into effective interface design.
Tue, 28 May 2024 - 05min - 490 - arxiv preprint - Octo: An Open-Source Generalist Robot Policy
In this episode, we discuss Octo: An Open-Source Generalist Robot Policy by Octo Model Team, Dibya Ghosh, Homer Walke, Karl Pertsch, Kevin Black, Oier Mees, Sudeep Dasari, Joey Hejna, Tobias Kreiman, Charles Xu, Jianlan Luo, You Liang Tan, Pannag Sanketi, Quan Vuong, Ted Xiao, Dorsa Sadigh, Chelsea Finn, Sergey Levine. The paper introduces Octo, a large transformer-based policy pretrained on 800k trajectories from the Open X-Embodiment dataset, designed to be a generalist policy for robotic manipulation. Octo can be instructed via language commands or goal images and can be efficiently finetuned to new sensory inputs and action spaces on various robotic platforms. Experimental results demonstrate Octo's versatility across 9 different robotic platforms and provide detailed analyses to guide future development of generalist robot models.
Fri, 24 May 2024 - 05min - 489 - arxiv preprint - Layer-Condensed KV Cache for Efficient Inference of Large Language Models
In this episode, we discuss Layer-Condensed KV Cache for Efficient Inference of Large Language Models by Haoyi Wu, Kewei Tu. The paper addresses the significant memory consumption issue in deploying large language models by proposing a novel method that computes and caches key-value pairs for only a small number of layers, thereby saving memory and enhancing inference throughput. Experiments demonstrate that this approach achieves up to 26× higher throughput compared to standard transformers while maintaining competitive performance. Additionally, the method can be integrated with existing memory-saving techniques for further efficiency improvements.
Thu, 23 May 2024 - 05min - 488 - arxiv preprint - Observational Scaling Laws and the Predictability of Language Model Performance
In this episode, we discuss Observational Scaling Laws and the Predictability of Language Model Performance by Yangjun Ruan, Chris J. Maddison, Tatsunori Hashimoto. The paper introduces an observational approach to building scaling laws for language models by utilizing approximately 80 publicly available models, bypassing the need for extensive model training. It discovers that despite variations in model efficiencies, performance can be predicted using a generalized scaling law based on a low-dimensional capability space. This method demonstrates the predictability of complex scaling behaviors and the impact of interventions such as Chain-of-Thought and Self-Consistency.
Wed, 22 May 2024 - 02min - 487 - arxiv preprint - Pack of LLMs: Model Fusion at Test-Time via Perplexity Optimization
In this episode, we discuss Pack of LLMs: Model Fusion at Test-Time via Perplexity Optimization by Costas Mavromatis, Petros Karypis, George Karypis. The paper presents PackLLM, a method for fusing knowledge from multiple Large Language Models (LLMs) during test-time by optimizing the importance of each LLM based on the input prompt to minimize perplexity. It introduces two variants: PackLLMsim, which validates perplexity as an expertise indicator, and PackLLMopt, which uses a greedy algorithm for perplexity minimization. Experiments with over 100 LLMs show that PackLLM outperforms existing test-time fusion approaches and learning-based fusers, demonstrating significant accuracy improvements.
Tue, 21 May 2024 - 04min - 486 - arxiv preprint - The Platonic Representation Hypothesis
In this episode, we discuss The Platonic Representation Hypothesis by Minyoung Huh, Brian Cheung, Tongzhou Wang, Phillip Isola. The paper argues that representations in AI models, particularly deep networks, are converging across various domains and data modalities. This convergence suggests a movement towards a shared statistical model of reality, termed the "platonic representation." The authors explore selective pressures driving this trend and discuss its implications, limitations, and counterexamples.
Mon, 20 May 2024 - 05min - 485 - arxiv preprint - Many-Shot In-Context Learning in Multimodal Foundation Models
In this episode, we discuss Many-Shot In-Context Learning in Multimodal Foundation Models by Yixing Jiang, Jeremy Irvin, Ji Hun Wang, Muhammad Ahmed Chaudhry, Jonathan H. Chen, Andrew Y. Ng. The paper examines the effectiveness of increased example capacities in multimodal foundation models' context windows to advance in-context learning (ICL). It specifically looks at the transition from few-shot to many-shot ICL, studying the impact of this scale-up using different datasets across various domains and tasks. Key findings reveal that using up to 2000 multimodal examples significantly boosts performance, indicating the potential of many-shot ICL in enhancing model adaptability for new applications and improving efficiency, with specific reference to better results from Gemini 1.5 Pro compared to GPT-4o.
Fri, 17 May 2024 - 03min - 484 - arxiv preprint - Naturalistic Music Decoding from EEG Data via Latent Diffusion Models
In this episode, we discuss Naturalistic Music Decoding from EEG Data via Latent Diffusion Models by Emilian Postolache, Natalia Polouliakh, Hiroaki Kitano, Akima Connelly, Emanuele Rodolà, Taketo Akama. The paper explores the use of latent diffusion models to decode complex musical compositions from EEG data, focusing on music that includes varied instruments and vocal harmonics. The researchers implemented an end-to-end training method directly on raw EEG without manual preprocessing, using the NMED-T dataset and new neural embedding-based metrics for assessment. This research demonstrates the potential of EEG data in reconstructing intricate auditory information, contributing significantly to advancements in neural decoding and brain-computer interface technology.
Thu, 16 May 2024 - 03min - 483 - arxiv preprint - The Chosen One: Consistent Characters in Text-to-Image Diffusion Models
In this episode, we discuss The Chosen One: Consistent Characters in Text-to-Image Diffusion Models by Omri Avrahami, Amir Hertz, Yael Vinker, Moab Arar, Shlomi Fruchter, Ohad Fried, Daniel Cohen-Or, Dani Lischinski. The paper introduces a novel method for creating character images that remain consistent in various settings using text-to-image diffusion models. It details a technique that extracts and maintains distinctive character traits from textual descriptions to achieve uniformity in visual representations. These consistent traits help in recognizing the character across varied backgrounds and activities in the generated images.
Wed, 15 May 2024 - 03min - 482 - arxiv preprint - Memory Mosaics
In this episode, we discuss Memory Mosaics by Jianyu Zhang, Niklas Nolte, Ranajoy Sadhukhan, Beidi Chen, Léon Bottou. Memory Mosaics are collective networks designed for prediction tasks, utilizing associative memories in a collaborative manner. These networks offer a simpler and more transparent alternative to transformers, maintaining comparable abilities in compositional learning and learning in context. The effectiveness of Memory Mosaics is established through medium-scale language modeling experiments, outperforming or matching the performance of transformers.
Tue, 14 May 2024 - 03min - 481 - arxiv preprint - Does Fine-Tuning LLMs on New Knowledge Encourage Hallucinations?
In this episode, we discuss Does Fine-Tuning LLMs on New Knowledge Encourage Hallucinations? by Zorik Gekhman, Gal Yona, Roee Aharoni, Matan Eyal, Amir Feder, Roi Reichart, Jonathan Herzig. The paper explores the effects of integrating new factual information into large language models (LLMs) during the fine-tuning phase, particularly focusing on how this affects their ability to retain and utilize pre-existing knowledge. It was found that LLMs struggle to learn new facts during fine-tuning, indicating a slower learning curve for new information compared to familiar content from their training data. Additionally, the study reveals that as LLMs incorporate new facts, they are more prone to generating factually incorrect or "hallucinated" responses, suggesting a trade-off between knowledge integration and accuracy.
Mon, 13 May 2024 - 03min - 480 - arxiv preprint - LongLoRA: Efficient Fine-tuning of Long-Context Large Language Models
In this episode, we discuss LongLoRA: Efficient Fine-tuning of Long-Context Large Language Models by Yukang Chen, Shengju Qian, Haotian Tang, Xin Lai, Zhijian Liu, Song Han, Jiaya Jia. The abstract describes "LongLoRA," a technique designed to efficiently expand the context size of large language models (LLMs) while maintaining computational feasibility. This methodology includes a novel "shifted sparse attention" mechanism and an improved Low-Rank Adaptation process for resource-efficient fine-tuning. It has been successfully tested on various tasks, offering increased context without requiring changes to the original model architecture, and is supported by openly available resources including the LongAlpaca dataset.
Fri, 10 May 2024 - 03min - 479 - arxiv preprint - WildChat: 1M ChatGPT Interaction Logs in the Wild
In this episode, we discuss WildChat: 1M ChatGPT Interaction Logs in the Wild by Wenting Zhao, Xiang Ren, Jack Hessel, Claire Cardie, Yejin Choi, Yuntian Deng. WILDCHAT is a dataset featuring 1 million user-ChatGPT conversations with over 2.5 million interaction turns, created by collecting chat transcripts and request headers from users who consented to participate. It surpasses other datasets in terms of diversity of prompts, languages covered, and the inclusion of toxic interaction cases, providing a comprehensive resource for studying chatbot interactions. Additionally, it incorporates detailed demographic data and timestamps, making it valuable for analyzing varying user behaviors across regions and times, and for training instruction-following models under AI2 ImpACT Licenses.
Thu, 09 May 2024 - 02min - 478 - arxiv preprint - Same Task, More Tokens: the Impact of Input Length on the Reasoning Performance of Large Language Models
In this episode, we discuss Same Task, More Tokens: the Impact of Input Length on the Reasoning Performance of Large Language Models by Mosh Levy, Alon Jacoby, Yoav Goldberg. The paper explores how the reasoning abilities of Large Language Models (LLMs) are impacted by increasing input lengths, utilizing a specialized QA reasoning framework to examine how performance is influenced by various input sizes. The findings reveal a noticeable drop in performance occurring at shorter input lengths than the maximum specified limits of the models, and across different datasets. It further points out the discrepancy between the models' performance on reasoning tasks with long inputs and the traditional perplexity metrics, suggesting opportunities for further research to overcome these limitations.
Wed, 08 May 2024 - 03min - 477 - arxiv preprint - NOLA: Compressing LoRA using Linear Combination of Random Basis
In this episode, we discuss NOLA: Compressing LoRA using Linear Combination of Random Basis by Soroush Abbasi Koohpayegani, KL Navaneet, Parsa Nooralinejad, Soheil Kolouri, Hamed Pirsiavash. The paper introduces a novel technique called NOLA for fine-tuning and deploying large language models (LLMs) like GPT-3 more efficiently by addressing the limitations of existing Low-Rank Adaptation (LoRA) methods. NOLA enhances parameter efficiency by re-parameterizing the low-rank matrices used in LoRA through linear combinations of randomly generated bases, allowing optimization of only the coefficients rather than the entire matrix. The evaluation of NOLA using models like GPT-2 and LLaMA-2 demonstrates comparable performance to LoRA but with significantly fewer parameters, making it more practical for diverse applications.
Tue, 07 May 2024 - 03min - 476 - arxiv preprint - StoryDiffusion: Consistent Self-Attention for Long-Range Image and Video Generation
In this episode, we discuss StoryDiffusion: Consistent Self-Attention for Long-Range Image and Video Generation by Yupeng Zhou, Daquan Zhou, Ming-Ming Cheng, Jiashi Feng, Qibin Hou. The paper introduces advanced techniques to improve diffusion-based generative models used for creating consistent and continuous sequences in image and video generation. It presents "Consistent Self-Attention" for maintaining content consistency and a "Semantic Motion Predictor" that aids in generating coherent long-range video content by managing motion prediction. These enhancements, encapsulated in the StoryDiffusion framework, allow for the generation of detailed, coherent visual narratives from textual stories, demonstrating the potential to significantly advance visual content creation.
Mon, 06 May 2024 - 03min - 475 - arxiv preprint - Iterative Reasoning Preference Optimization
In this episode, we discuss Iterative Reasoning Preference Optimization by Richard Yuanzhe Pang, Weizhe Yuan, Kyunghyun Cho, He He, Sainbayar Sukhbaatar, Jason Weston. This study explores a new iterative method aimed at improving how AI models generate step-by-step logical reasoning, or Chain-of-Thought (CoT), to reach correct answers by optimizing between competing reasoning steps. The technique uses a specialized loss function, incorporating negative log-likelihood, to systematically refine the reasoning accuracy of AI responses. It has been tested on a Llama-2-70B-Chat model and demonstrated significant performance improvements across different reasoning benchmarks without the need for additional external data.
Fri, 03 May 2024 - 03min - 474 - arxiv preprint - Better & Faster Large Language Models via Multi-token Prediction
In this episode, we discuss Better & Faster Large Language Models via Multi-token Prediction by Fabian Gloeckle, Badr Youbi Idrissi, Baptiste Rozière, David Lopez-Paz, Gabriel Synnaeve. The paper "Better & Faster Large Language Models via Multi-token Prediction" introduces a novel training methodology for large language models (LLMs) by predicting multiple future tokens simultaneously rather than the traditional single next-token prediction. This technique utilizes multiple independent output heads on a shared model trunk to predict several tokens at once, enhancing sample efficiency and model performance on generative tasks without increasing training times. The models trained using this method not only show improved results in tasks like coding but also benefit from faster inference times, up to three times quicker than traditional models.
Thu, 02 May 2024 - 03min - 473 - arxiv preprint - Make Your LLM Fully Utilize the Context
In this episode, we discuss Make Your LLM Fully Utilize the Context by Shengnan An, Zexiong Ma, Zeqi Lin, Nanning Zheng, Jian-Guang Lou. The paper "Make Your LLM Fully Utilize the Context" delves into solving the lost-in-the-middle challenge in large language models (LLMs), where these models fail to fully use the contextual information provided in longer texts. The authors introduce a new training technique called INformation-INtensive (IN2) aiming to enhance processing and integration of detailed information across extensive text segments up to 32,000 tokens. They implement this method in a model called FILM-7B (FILl-in-the-Middle), demonstrating its superior ability to handle long-context scenarios effectively alongside maintaining performance on shorter contexts, and showing significant improvements in tasks such as NarrativeQA.
Wed, 01 May 2024 - 03min
Podcast simili a <nome>
Global News Podcast BBC World Service
El Partidazo de COPE COPE
Herrera en COPE COPE
Tiempo de Juego COPE
The Dan Bongino Show Cumulus Podcast Network | Dan Bongino
Es la Mañana de Federico esRadio
La Noche de Dieter esRadio
Hondelatte Raconte - Christophe Hondelatte Europe 1
Affaires sensibles France Inter
La rosa de los vientos OndaCero
Más de uno OndaCero
La Zanzara Radio 24
Les Grosses Têtes RTL
L'Heure Du Crime RTL
El Larguero SER Podcast
Nadie Sabe Nada SER Podcast
SER Historia SER Podcast
Todo Concostrina SER Podcast
安住紳一郎の日曜天国 TBS RADIO
TED Talks Daily TED
The Tucker Carlson Show Tucker Carlson Network
辛坊治郎 ズーム そこまで言うか! ニッポン放送
飯田浩司のOK! Cozy up! Podcast ニッポン放送
武田鉄矢・今朝の三枚おろし 文化放送PodcastQR